Machine Intelligence: Building Your Own or as‑a‑Service
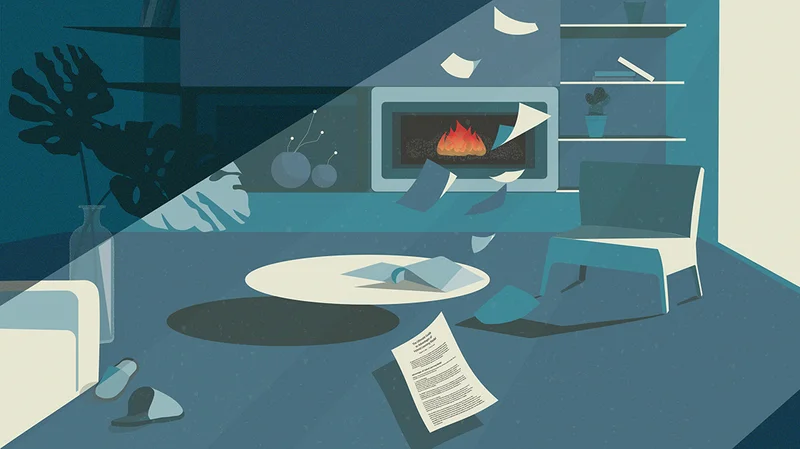
Machine intelligence is on the up and businesses worldwide are facing the decision of when to implement and whether they will choose to develop in-house or look to third party providers.
Machine intelligence is starting to creep into the mainstream and we are now entering a time where businesses want to harness that intelligence to increase productivity and profitability. The need for digital ecosystems raises the question of when an organization should build its own MI or if they should outsource to a third party.
The key components of machine intelligence are:
- Computing strength
- Algorithms
- Data
If a company is strong in these areas then they are ready to build their own MI. If not, they will probably be better served accessing a third party who will provide it as a service. These strengths are not all about technology or budget, but also about how well equipped the workforce is to deal with building and implementing MI.
Computing Strength
In the past anything used for machine learning would require a super computer but with the advent of the cloud it is easier to harness the sort of power needed for MI solutions. Not only that but is has considerably lowered the cost and companies can simply rent what they need; as a result there are very few instances where building your own machine learning infrastructure is cost effective. That said, picking the right cloud infrastructure can made a big difference. Specialty hardware is needed so think about vetting providers; the type of service provided by Google and Microsoft is the gold standard.
Algorithms
If your company’s algorithms are better than others then using proprietary algorithms may be cost effective. However in-house building costs can soar and there needs to be a high level of workforce expertise. Assess any advantage your organization may have against the proprietary solution.
MI is likely to be increasing with many thousands of future apps being able to perform multiple functions. It may be more cost effective to invest in training staff in API management rather than focus on ML algorithms.
Data
Most companies are relatively strong in data and the actual content varies between organizations. Although computing infrastructure and algorithms lend well to commoditization, data is something that will probably remain in-house. More and more companies are now looking at ways to turn their data into funds and the use of ML is one way this can be achieved. As a result there has been a huge hike in demand for Data Scientists in the worldwide job market.
Over the last 10 years there have been multiple examples of new platforms, new ecosystems and new ways of building services and products. Google Maps is a key example of something that can be replicated and integrated into multiple services. Opportunities for companies to utilize these kinds of technology will continue to grow with machine learning and artificial intelligence playing a key role. There is a growing market for suppliers to provide ML as a service saving organizations millions of dollars in development costs and taking the strain away from valuable company resources.
If you’re looking for a company that provides PHP Symfony development and other software development services contact us.