What’s the difference between machine learning and deep learning?
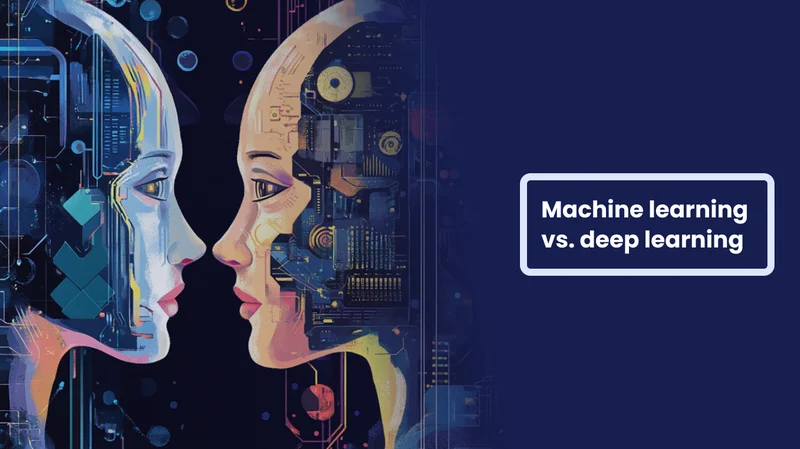
Can you tell the difference between machine learning and deep learning? If your answer was that one starts with the word ‘machine’ and the other has ‘deep,’ you’re not wrong. But this is not the full picture.
While many consider machine learning and deep learning interchangeable terms, they represent different approaches within AI. Traditionally, the distinction was clear: deep learning focused on layered neural networks, and machine learning covered a broader spectrum of algorithms. But as technology advances, where do we draw the line? Are these terms still meaningful, or has the gap between them blurred over time?
Let’s explore what makes these concepts stand apart, how they overlap, and whether comparing them still makes sense.
Machine learning
According to the Oxford Dictionary, machine learning is a type of artificial intelligence in which computers use huge amounts of data to learn how to do tasks rather than being programmed to do them. In short, machine learning is about training a computer to perform tasks without detailed instructions.
These systems use algorithms to process large amounts of data, find patterns, and make decisions and predictions based on the information they’ve analyzed. The more data they chew through, the better they become at making decisions. It’s a bit like teaching a child to ride a bike: the more they practice, the less likely they are to end up in a bush!
Three types of machine learning models
The learning process of these algorithms can be supervised, unsupervised, semi-supervised and reinforcement. It depends on the data that is used to feed the algorithms.
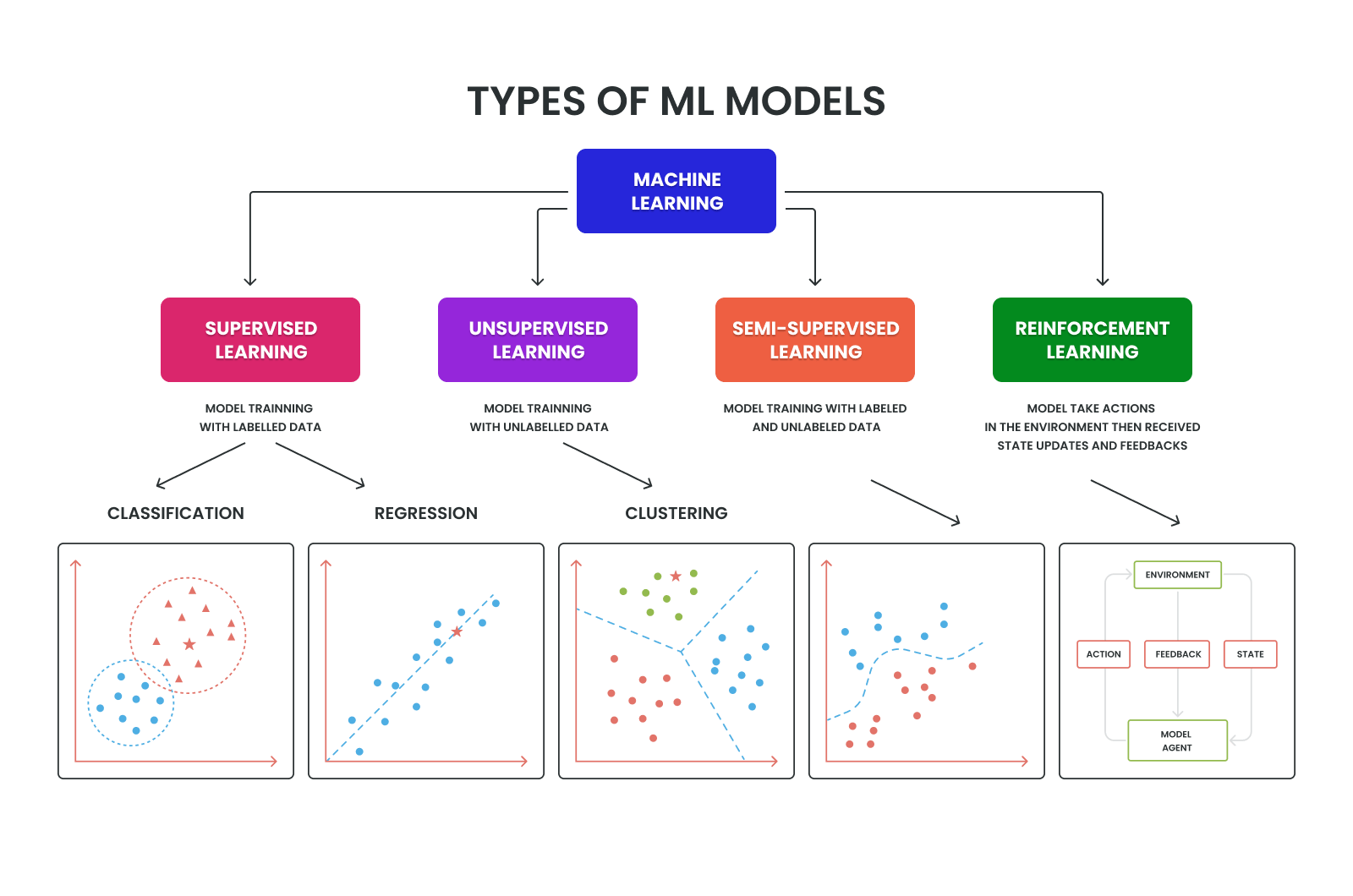
Types of ML models
Supervised learning uses labeled data to train a machine to match inputs to specific outputs. For example, to teach an algorithm to recognize apples, you give it labeled pictures of apples. The algorithm learns by using this known data.
When the output is unknown, we deal with unsupervised learning. As the name suggests, this works without human guidance, using unlabeled data to find patterns. The algorithm categorizes data on its own based on shared traits. For example, if you give it pictures of apples and bananas, it will figure out which is which without being told.
Semi-supervised learning combines labeled and unlabeled data. The labeled examples guide the algorithm while it learns patterns from the unlabeled ones. For instance, with a few labeled pictures of apples and bananas and many unlabeled images, the algorithm uses the labeled data to classify the rest.
Reinforcement learning works differently. The algorithm interacts with its environment and gets feedback — rewards or penalties. For example, in a game of chess, the algorithm learns to make moves that lead to winning the game over time. It receives positive feedback for good moves and negative feedback for poor choices. This helps to improve its strategy.
Spotify is a familiar example of a machine learning algorithm in action. It learns your music taste by tracking the songs you listen to or save. Each time you interact with a song, the algorithm adjusts to offer better recommendations next time.
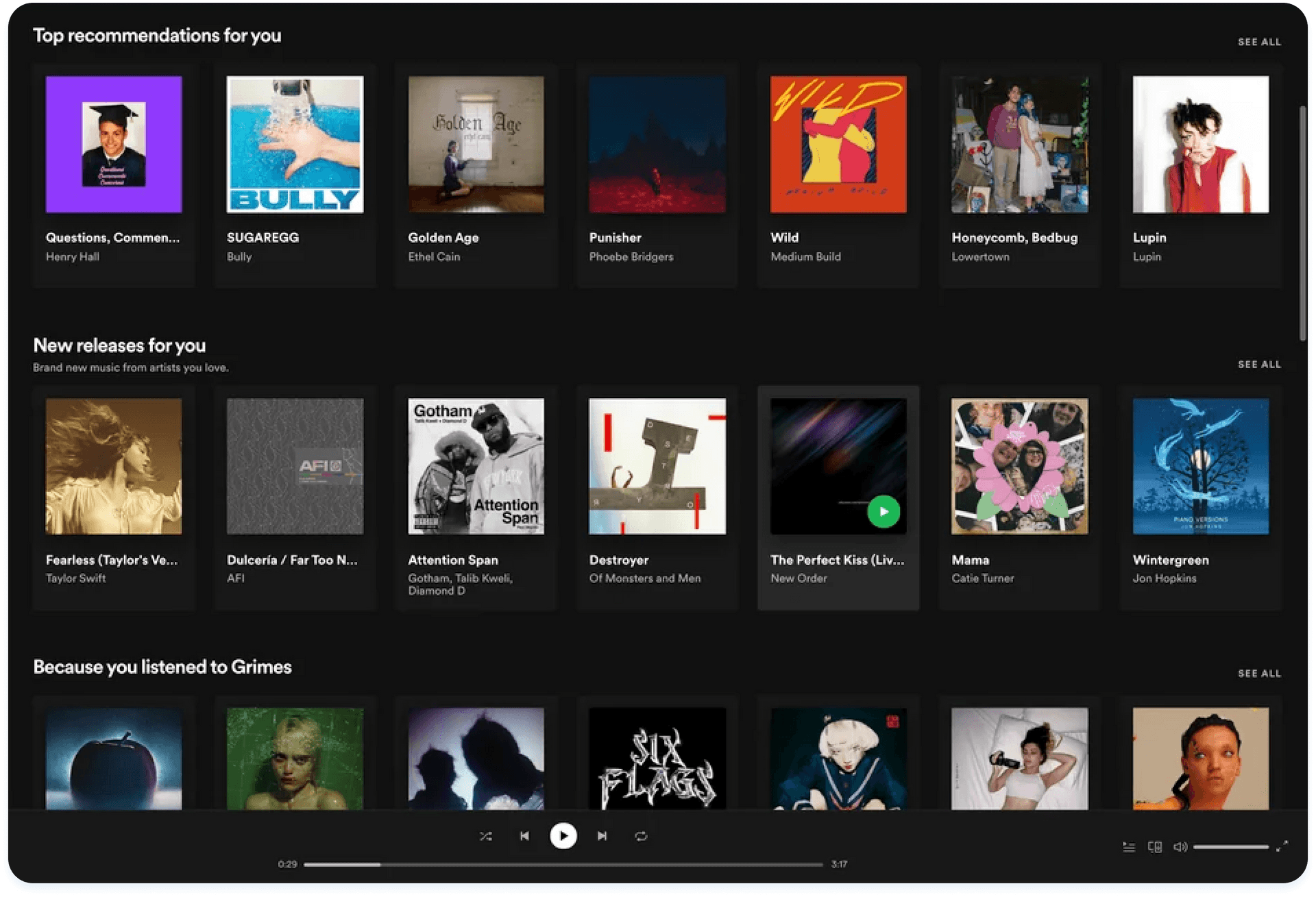
Recommendations in Spotify
Do you want to learn more about the ML model types?
Read our machine learning guide and discover how ML models work and how your company can benefit from it.
Deep learning – a sophisticated evolution of machine learning
Deep learning is a subset of machine learning, but much more sophisticated and mathematically complex. It takes machine learning to the next level, using neural networks modeled after the human brain. Imagine if your brain were on overdrive, crunching data at lightning speed – that’s deep learning!
While traditional ML algorithms often need human adjustments when they produce incorrect results, deep learning algorithms learn on their own through repetition, improving their performance without human intervention.
What is a neural network?
To understand how deep learning works, it’s essential to first define the term neural network. Neural networks, also known as artificial neural networks, are a fundamental part of deep learning algorithms. They are called “neural” because they mimic the way neurons in the brain communicate and learn.
- Neural networks are organized into layers: an input layer, one or more hidden layers, and an output layer.
- Each layer is made up of nodes, which function like artificial neurons.
- These nodes are interconnected, and each connection has a weight that determines the strength of the signal transmitted between them.
Additionally, each node has a threshold value that determines whether it activates based on the input it receives.

How does it work?
Input layer
The process begins at the input layer, where data enters the neural network. This layer consists of multiple nodes that receive raw data, which can be an image, text, or sound. Think of this as the front door of a house: it’s where everything comes in before it gets sorted out.
Hidden layers
Next are the hidden layers, where the real analysis occurs. Each hidden layer processes the information from the input layer, focusing on different features. For example, if the task is to identify an animal in an image, one layer might examine its size, while another looks at its fur pattern. This teamwork helps the network make better sense of the data.
Output layer
Finally, the output layer delivers the results. If the task is a simple yes or no question, like whether an image contains a cat, the output layer might have just two nodes.

Example of a fully connected neural network designed for binary classification
The best time to implement AI in your business is now!
Explore our AI consulting and development services and see how we can help you innovate and grow.
What made deep learning necessary?
Deep learning was created to reduce the need for constant human involvement in machine learning.
As we’ve stated before, traditional machine learning relies on humans to manually define features – a process called feature engineering. For example, if you’re teaching an ML model to recognize cats and dogs, you’d have to manually specify things like the shape of ears, tails, or noses.
Deep learning helps achieve this ultimate goal of ML – it cuts out the need to manually label data at every stage.
Although deep learning has been around for a while, it wasn’t widely used until the early 2000s. Back then, the problem wasn’t the theory – it was the lack of large datasets and affordable computing power. Fast forward 20 years, and with the rise of data and cheaper processing, deep learning has finally become a very popular practical solution for many businesses.
Deep learning – a term that’s losing its depth?
But the world doesn’t stand still. Technology evolves at lightning speed, and what seemed revolutionary yesterday can feel outdated today. This raises an important question: Is the term “deep learning” still relevant? Looking ahead, the answer may be no. Let’s explore why.
Historical context of “deep”
When AlexNet was introduced in 2012, its 8-layer architecture attracted significant attention and set a new standard in neural networks. When Google introduced its 22-layer network GoogLeNet back in 2014, it was groundbreaking. The term “deep learning” made perfect sense, as networks with such depth were once considered cutting-edge. But today, models with hundreds of layers are not uncommon, and building them is no longer a technical marvel. The very definition of “deep” has become blurry.
Blurred boundaries today
As the field advances, the exact definition of “deep” has become less relevant. The focus has shifted to designing architectures that are efficient and capable, regardless of their depth. For instance:
- Wide networks with fewer layers can outperform deeply stacked architectures in some scenarios.
- Techniques like transfer learning or pre-trained models (e.g., BERT, CLIP) rely on depth but don’t emphasize it explicitly in discussions.
Categories are evolving
Instead of focusing on layer counts, today’s discussions are more about types of architectures (e.g., convolutional, recurrent, transformer-based) or capabilities (like generalization, adaptability, or multi-modality). The “deep” qualifier may seem less meaningful in this broader context.
Take GPT-based systems like ChatGPT, for example. These models are built on massive neural network architectures – technically deep learning. Yet, they are rarely referred to as such in everyday discussions. Instead, the focus is on what these systems can do, like generating coherent text or solving complex problems, rather than how many layers they contain.
However, despite its technical boundaries becoming vague, “deep learning” remains widely recognized and understood by both technical and non-technical audiences. It’s a term with historical and practical significance that grounds discussions in AI development. For clarity and convenience, we continue to use it in this article to explain some more ideas.
What do ML and deep learning have in common?
From a bird’s-eye view, both ML and DL find patterns in data. They use datasets to train algorithms based on complex mathematics. During training, these algorithms learn how inputs relate to outputs, which helps them predict results from new data. This learning process mostly happens automatically unlike traditional programming.
Here are some more touch points:
- AI techniques. Both ML and DL belong to the broader data science and AI ecosystem.
- Application. They both excel at handling tasks that are too time-consuming – or outright impossible – with traditional programming approaches.
- High computational demand. Running ML algorithms needs some serious computing power, and deep learning is even hungrier for resources. Luckily, thanks to tech advancements, they’re now more accessible to everyone.
Learn how we helped an American retailer implement AI-based behavior analysis and sales forecasting
Thanks to our solution, the customer achieved a 7% increase in visitors-to-buyers conversion rate, a 15% rise in data volume collected, and a 35% reduction in monthly infrastructure costs!
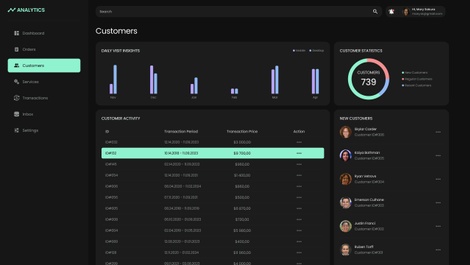
What are the key differences between ML and deep learning?
We’ve already highlighted the basics: deep learning goes beyond ML with its complex neural networks and reduced need for human input. While ML uses supervised, unsupervised, and reinforcement learning, deep learning processes data through multiple layers, needing far more computing power.
But beyond these, there are a few more differences that aren’t on the surface:
Type of task
The use of ML or DL depends on the challenge you’re addressing. Neural networks are particularly strong when it comes to processing and generating data like images, sounds, and videos, where they can deliver impressive results. However, simpler tasks may be more efficient with machine learning.
Interpretability
ML models are easier to explain. You can follow the logic in decision trees or regression models like a simple flowchart. With deep learning, it’s a bit like a “black box”—the more layers there are, the harder it is to figure out how it reached a conclusion.
Performance
Both ML and deep learning shine in different areas. For simpler tasks, like filtering spam emails, ML typically performs faster and more efficiently. But when it comes to more complex problems, like medical image analysis, deep learning takes the lead. Its ability to spot hidden patterns and details allows it to outperform ML in tasks requiring higher accuracy.
However, deep learning models, particularly neural networks, can be difficult to maintain in production. They are highly sensitive to changes and data drifts, which can cause their performance to degrade over time. In contrast, machine learning models tend to be more stable and reliable in production environments.
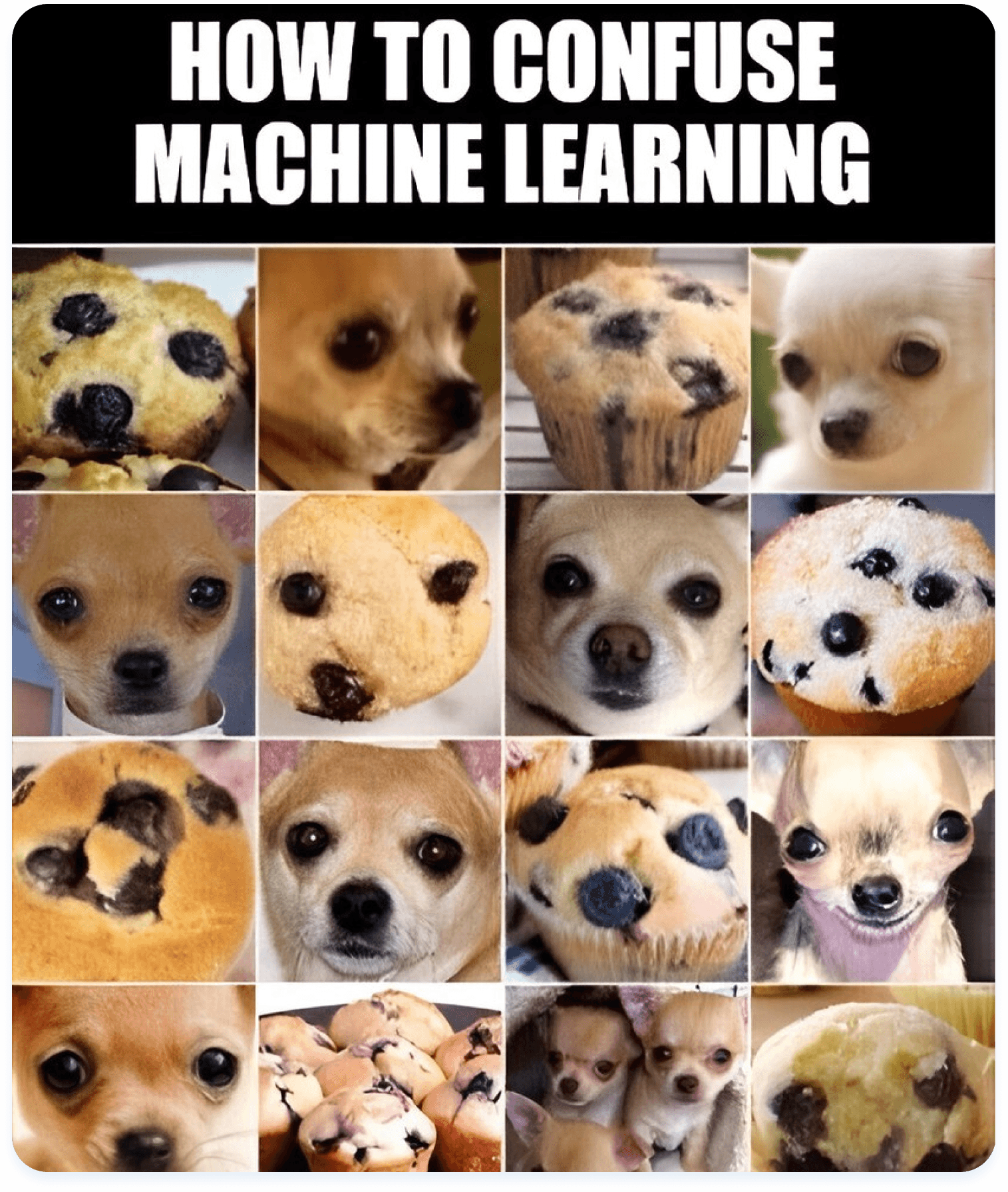
Let’s summarize!
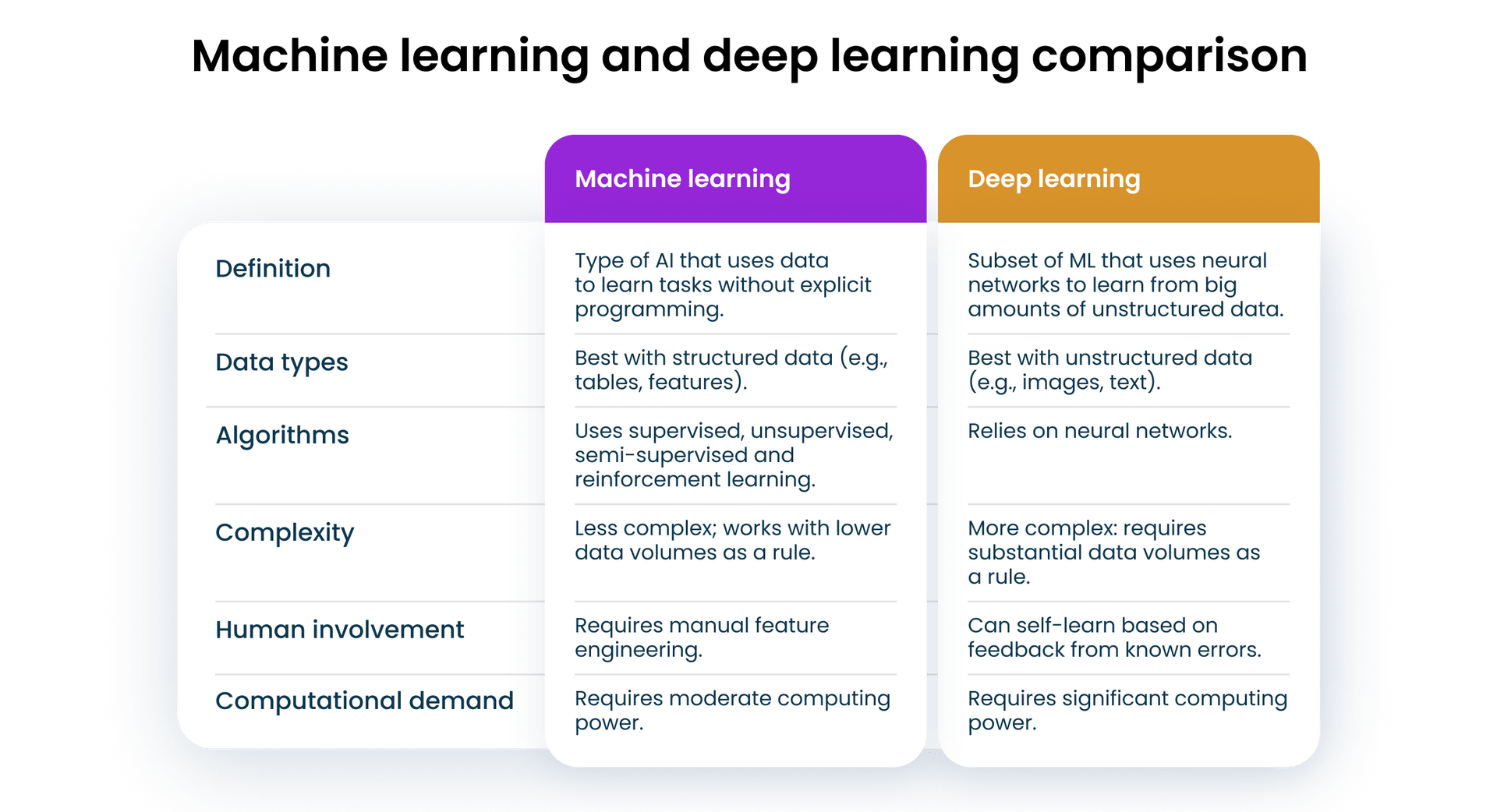
Machine learning and deep learning comparison
Get started with machine learning
Have you already chosen the right technology for your business? If not, Aristek is here to help. As part of our ML consulting services, we will analyze your business challenges and needs, audit your data, and recommend the best solution tailored to you. If you’ve already made your choice, our skilled engineers are ready to assist with the development and implementation process.
Book a free consultation, share your thoughts, and let’s get started!