How AI Can Drive Innovation in Healthcare
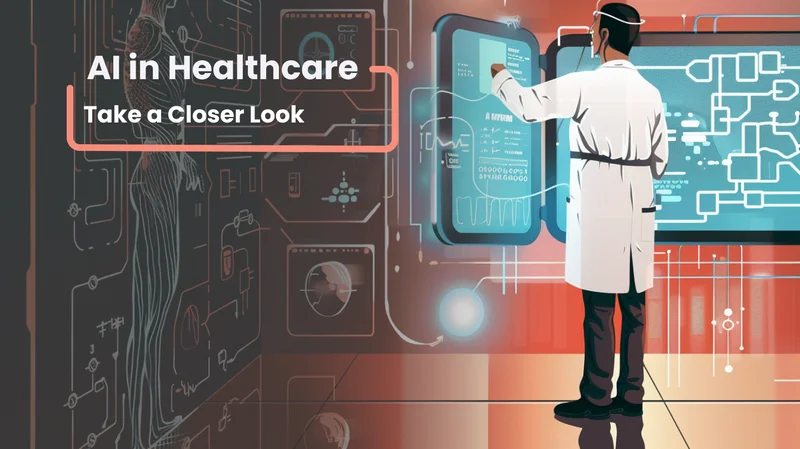
For the best possible insights, we’ve combed through various scientific studies, examined plenty of statistics, and talked to experts in the field.
Hold on tight. This will be a captivating read.
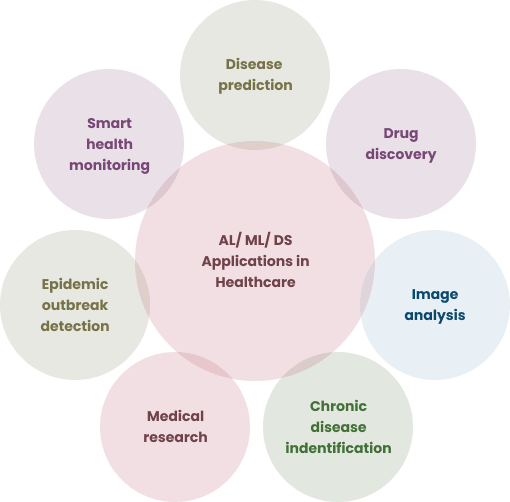
AI Applications in Healthcare
Yet, six in ten Americans say they would be uncomfortable if their healthcare provider relied on AI to treat them.
Clearly, questions arise.
- Is there anything to worry about, at least for the moment?
- Can AI be truly helpful in treatment?
- And how is it actually used today?
We’ll assess AI efficiency in healthcare using two criteria:
- Theory: research findings, AI prospects, and potential;
- Practice: real-world use cases, tangible benefits, and current challenges.
Diagnosis & Treatment
Today, many tech companies are developing algorithms for interpreting images and big data prediction models.
Their mission is to create “a co-pilot” for clinicians to assist with diagnosis and treatment decisions.
Theory
Researchers are now claiming that their AI-based approach can diagnose and treat diseases as accurately as a human clinician. Or even better.
Studies have shown AI’s ability to meet or exceed human experts’ performance in image-based diagnoses in radiology, dermatology, pathology, and cardiology.
For example, a study published in the UK used an AI system to diagnose breast cancer based on a large dataset of mammograms.
It showed that an AI-based mammogram interpretation system decreased false positive and negative results, which will potentially improve accuracy of breast cancer screening.
Another research used artificial intelligence to detect skin cancer.
It showed that AI technology had a 100% detection rate for melanoma and was 99.5% accurate in detecting all skin cancers.
Providers and payers for care also use ‘population health’ ML models to predict populations at risk of particular diseases or accidents.
These models can be effective, but they sometimes lack relevant data that adds predictive capability.
For instance, patient socio-economic status.
Unlock the Promise of Healthcare with Customizable AI
Innovate diagnosis, treatment, and workflow with customizable AI solutions tailored for your health system’s needs – connect with our experts to evaluate implementation options.
Practice
Indeed, automated classification of medical images proves to be useful in diagnostics and treatment.
In fact, it is the leading AI application today.
A recent review of AI/ ML-based medical devices approved in the USA and Europe from 2015 to 2020 found that 58% of devices in the USA and 53% in Europe were approved or CE marked for radiological use.
Let’s see how it works:
Imagine a computer tomography (CT) scan.
AI can find a very similar scan and tell the dynamics of changes based on these two pictures.
Now, instead of a few experts interpreting a scan, picture ten million radiologists doing so.
That’s basically how AI uses medical imaging to help reduce diagnostic mistakes by detecting anomalies a human might overlook.
What’s more, AI can learn from a wealth of data.
An AI tool that has learned all the ways a disease can present itself in an imaging study can quickly derive answers from new imaging studies.
And all this without getting tired.
A good example of a platform that uses AI is Nuance’s Precision Imaging Network. It interprets pictures and provides radiologists with recommendations.
We must say, recommendations is a keyword here.
Today, AI doesn’t produce an independent prognosis. No medical institutions fully delegate diagnostics to AI to avoid lawsuits.
Strictly speaking, it is used for more accurate measurements and highlighting spots potentially useful for the doctor.
There are more challenges to using AI in healthcare.
According to the scientific report, AI implementation issues are an uphill struggle for many healthcare organizations.
This situation is beginning to change, but AI is mostly present in research labs and in tech firms rather than in clinical practice.
Another challenge for AI adoption in healthcare is ensuring widespread use.
This requires regulatory approval, integration with EHR systems, standardization, clinician training, funding, and field updates.
Therefore, scientists claim that AI’s maturity will take longer, resulting in limited use within 5 years and more extensive use within 10.
Administrative Applications
AI can optimize administrative tasks in all kinds of industries. No wonder, this kind of application is popular.
Theory
According to McKinsey, GenAI represents a meaningful new tool that can help unlock a piece of the unrealized $1 trillion of improvement potential present in the industry.
“It can do so by automating tedious and error-prone operational work, bringing years of clinical data to a clinician’s fingertips in seconds, and by modernizing health systems infrastructure.”
These are needed in healthcare because, for example, the average US nurse spends 25% of work time on regulatory and administrative activities.
Practice
This is the case where theory actually corresponds to the practice.
Here is how AI is used to optimize administrative tasks.
RPA (Robotic Process Automation) platforms are used for revenue cycle management, clinical recording, claims processing, medical record administration, and continuity care.
For example, they summarize discharge information and follow-up needs for post-acute care.They can also generate care summaries for referrals, and synthesize specialist notes for the primary-care physician team.
Machine learning is used in claims and payment administration for probabilistic data matching across databases.
As insurers have a duty to verify whether the millions of claims are correct, ML helps identify and correct issues faster and more effectively.
As a result, it saves all stakeholders (health insurers, governments and providers) a great deal of time, money and effort.
NLP systems are primarily used for clinical documentation and research.
Speech and text recognition are increasingly utilized in healthcare for tasks like patient communication and clinical note capture.
Patient Engagement
One of the most promising but tricky AI uses in healthcare is patient engagement and communication.
Theory
At present, AI-based capabilities are being explored for personalized care, with a focus on machine learning and business rule engines for targeted interventions and timely action.
The main question is: can AI personalize and contextualize care?
Can it be achieved by using messaging alerts and targeted content that provoke actions at moments? That’s an intriguing field of research.
Another major focus is guiding patient behavior in a more anticipatory manner using real-world evidence. This is how it looks:
- Software collects information from provider EHR systems, biosensors, wearables, cell phones, etc;
- Then compares patient data to previous effective treatment routes for similar cohorts;
- Finally, it adapts suggestions based on all of the above.
The advice might be given to doctors, patients, nurses, call center representatives, or care delivery coordinators.
This leads us to virtual health assistants.
Virtual assistant is an innovative technology designed to simulate human conversation and offer personalized patient care based on input from the patient.
According to research, virtual assistants use AI-powered applications, chatbots, sounds, and interfaces.
They help patients to identify the problem based on the symptoms, provide medical advice, remind patients to take their medications, schedule doctor appointments, and monitor vital signs.
Practice
AI chatbots (e.g. Babylon and Ada) can identify symptoms and recommend further actions in community and primary care settings.
They can also be integrated with wearable devices like smartwatches to help patients and caregivers improve their behavior, sleep, and overall well-being.
For example, Emerald is a wireless sensor and ML platform for remote monitoring of sleep, breathing, and behavior.
It was founded by Massachusetts Institute of Technology faculty and researchers.
Yet, let’s remember that AI chatbots don’t provide you with a diagnosis.
Their suggestions only have a recommendation character, which they constantly remind us of.
Some healthcare organizations experiment with chatbots for patient interaction, mental health and wellness, and telehealth.
However, in a survey of 500 US users of the top five chatbots used in healthcare, patients expressed concern about revealing confidential information, discussing complex health conditions, and poor usability.
Surgeries
This is a sphere where AI has proven to be highly effective.
For this purpose, we won’t even examine theory and practice separately.
First surgical robots were approved in the USA in 2000.
This technology provides ‘superpowers’ to surgeons, improving their ability to see and create precise and minimally invasive incisions.
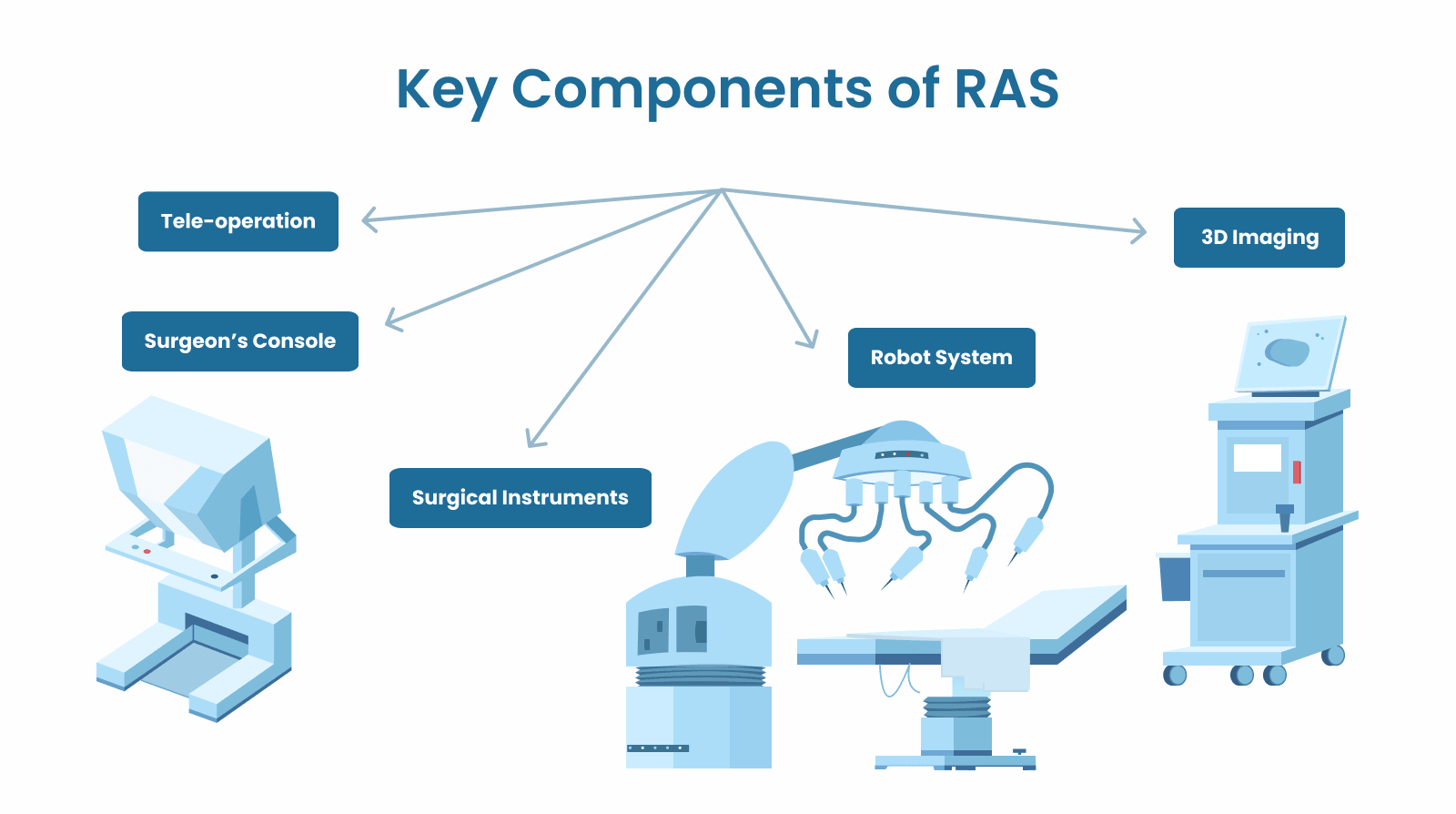
Robot-Assisted Surgery Components
Common procedures performed using robots include gynecologic, prostate, head, and neck surgeries.
Ryan Cameron, vice president of technology and innovation at Children’s Hospital & Medical Center in Nebraska says:
“We do a high volume of laparoscopic surgeries using the da Vinci Surgical System. It’s hard for a person to achieve perfect stillness because of breathing and heart rate. The da Vinci system absorbs the surgeon’s natural body movements and makes sure everything happens with great precision.”
However, important decisions are still made by human surgeons.
Some more great uses of AI include 3D organ reconstruction for surgeons to plan operations and computer vision during surgeries to evaluate surgery control.
Drug Discovery & New Therapies
Whether AI can find new treatments is one of the most puzzling questions.
Let’s see what the current situation is.
Theory
AI is expected to drive significant improvement in clinical trial design and optimization of drug manufacturing processes.
The recent announcements by DeepMind and AlphaFold pave the way for better understanding disease processes, predicting protein structures, and developing more targeted therapeutics.
AI tools on multimodal datasets could enhance disease understanding and clustering, as well as patient population clustering.
It will enable targeted preventive strategies like immunomics for diagnosis and treatment prediction.
This will be revolutionary for multiple standards of care, with particular impact in the cancer, neurological, and rare disease spaces. As a result, personalizing the experience of care for the individual.
Practice
Over the past decade, synthetic biology has produced developments like CRISPR gene editing and some personalized cancer therapies.
Yet, the creation process for such sophisticated treatments is still incredibly costly and inefficient.
In the future, AI will enable us to handle far more systematic complexity and, consequently, help us transform the way we understand, discover, and affect biology.
This will be made possible by improved access to various data.
The efficiency of the drug discovery process is expected to improve by helping better predict early which agents are more likely to be effective, and anticipate adverse drug effects.
That is a critical point because these issues have stymied the development of effective drugs at a costly late stage in the development process.
As a result, we can expect more democratic access to advanced therapies at a lower cost.
Key Points
AI healthcare research is accelerating rapidly. Potential use cases in healthcare include drug discovery, virtual assistants, disease diagnosis and treatment, health monitoring, and many others.
Currently, AI systems can’t reason the same way as human physicians. Instead, AI resembles a signal translator, translating patterns from datasets.
AI systems today are beginning to be adopted by healthcare organizations to automate time consuming, high volume repetitive tasks. They can also be used only to help doctors during surgeries or assist with diagnostics.
Yet, they don’t diagnose independently. The same works for AI apps whose predictions have only a recommendation character.
This is the current situation with AI in healthcare. But forecasts are very promising. More extensive use is expected in the next ten years.
Polaris Market Research predicts AI in the healthcare market will be worth $431 billion by 2032.
If you are considering boosting your business with AI, now is the perfect time.