What happens when data science meets retail?
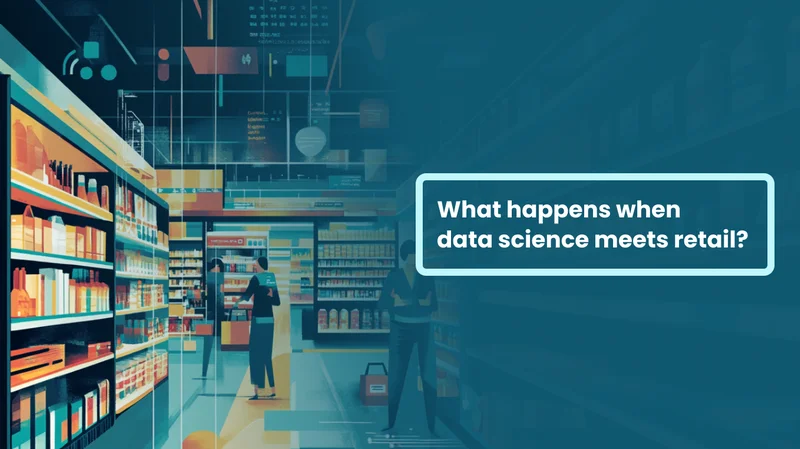
This article will introduce you to data science (DS), its applications in the retail industry, benefits and implementation challenges, and ways to deal with them. Let’s get started!
What is data science (DS)?
First of all, let’s get the gist.
Data science (DS) involves using various techniques and tools to analyze and interpret complex datasets. Its goal is to extract knowledge from data to help make informed decisions.
Basically, DS is all about finding insights in data.
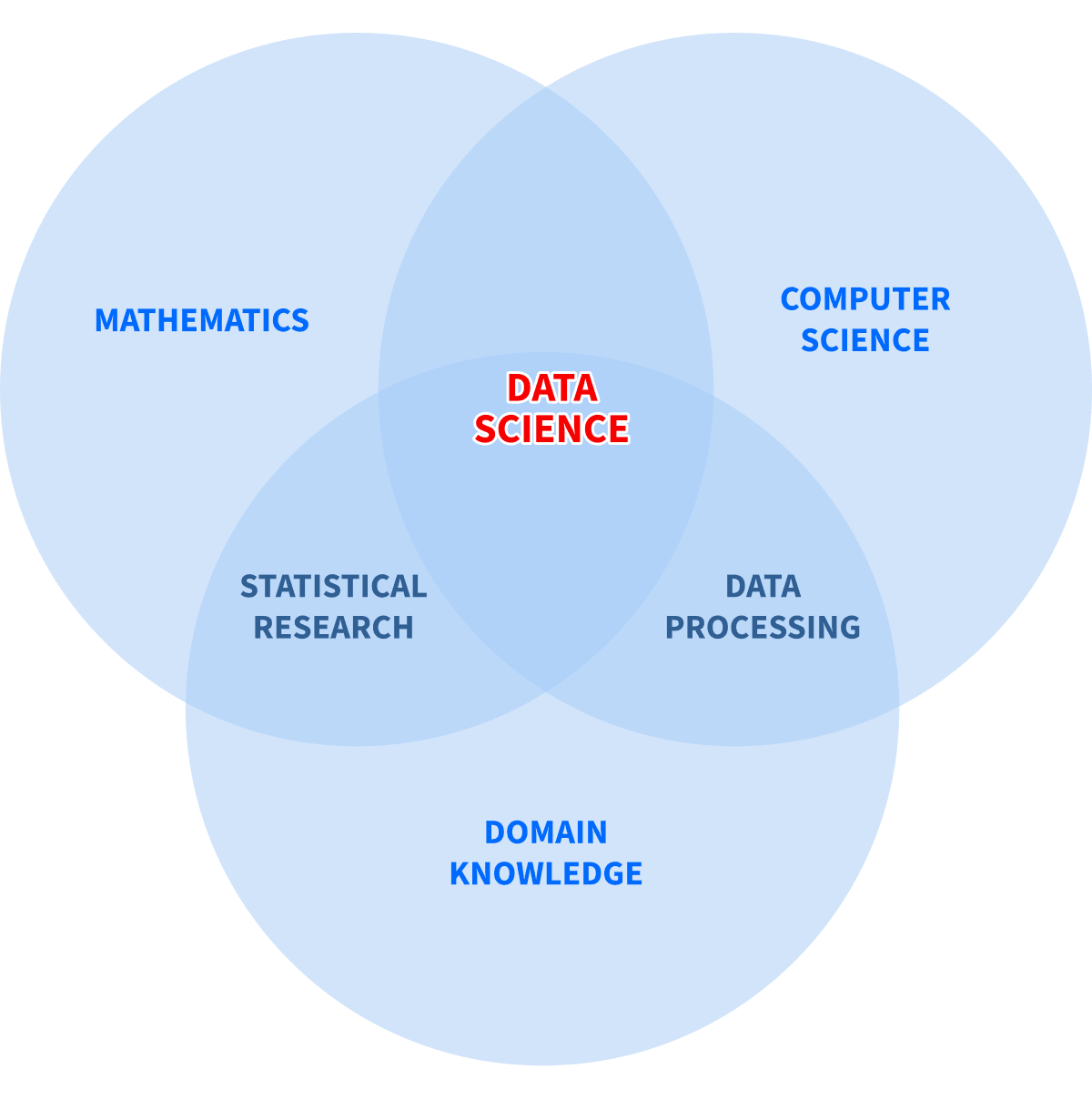
Data science concept
Is DS the same thing as AI? Well, let’s see.
Data science stands at the crossroads of artificial intelligence (AI) and statistics. So, not all AI is DS, but there’s a huge overlap.
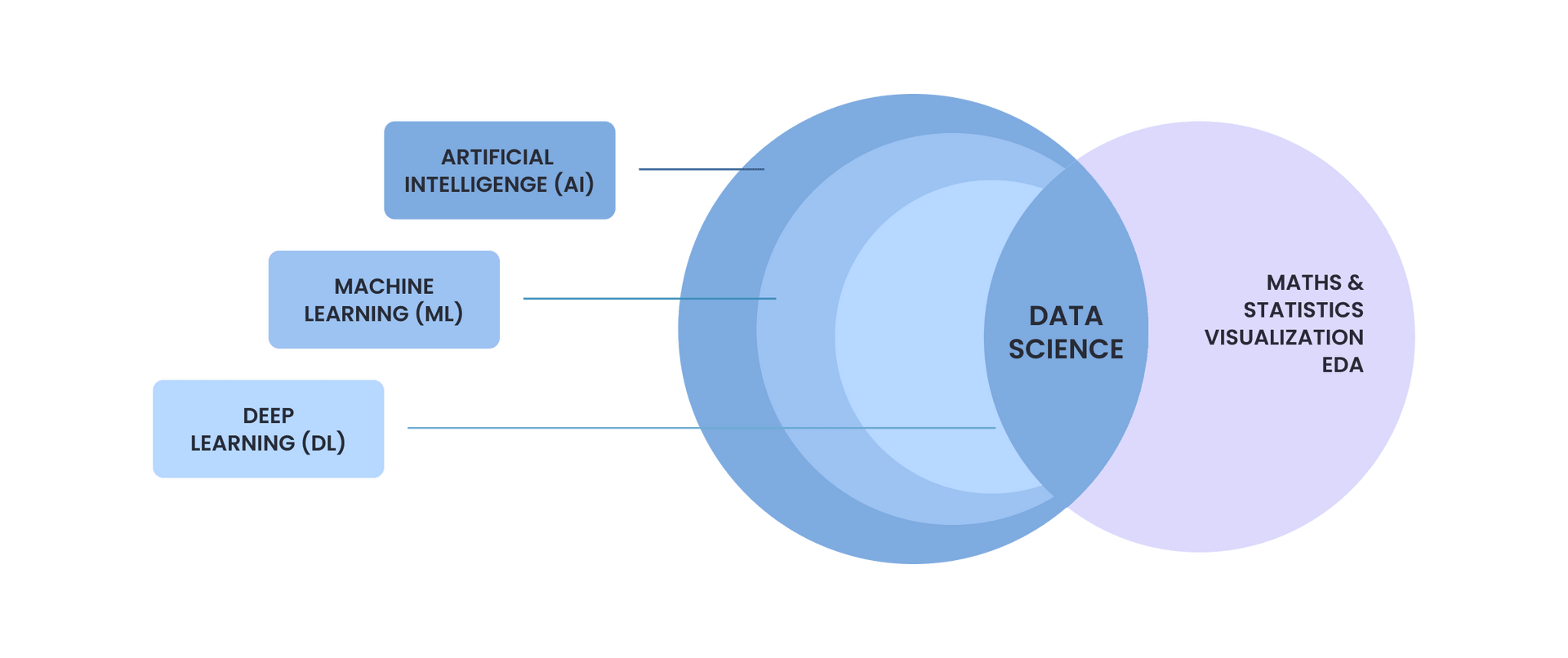
DS & AI overlap
AI = DS, when it’s used to gain insights. Therefore, all predictive analytics and recommendation systems are data science.
But much of AI stuff isn’t DS. For example, speech recognition, natural language processing (NLP), robotics, etc.
The same way, DS isn’t always about AI. For instance, you can do some basic data science even in MS Excel.
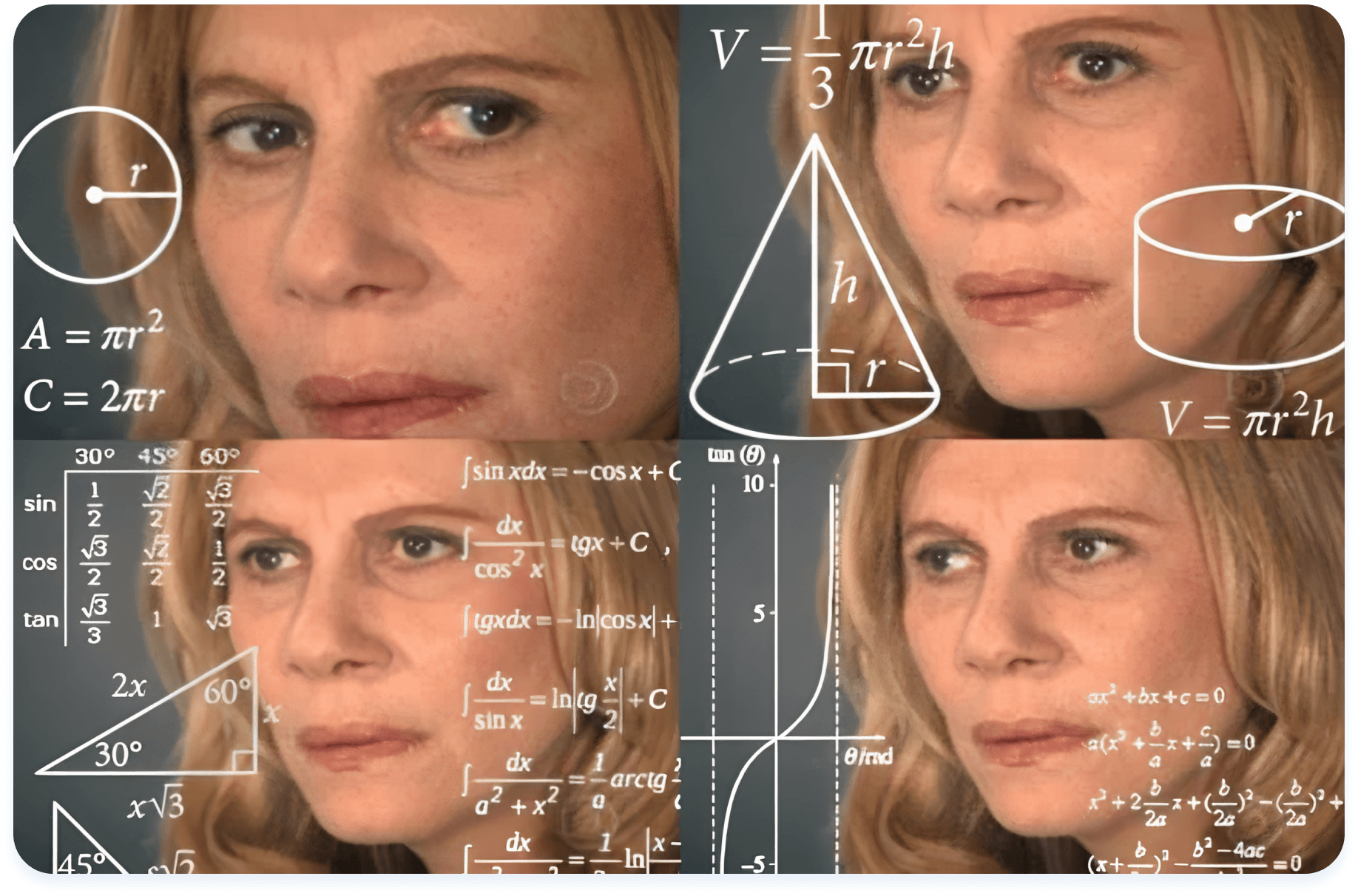
Let’s sum this up:
Generating cat images is not data science, but generating a sales report is.
Data science & retail
The global data science market is increasingly growing, and it’s projected to grow at a CAGR of 15.6% from 2023 to 2027.
This is because data science helps multiple industries gain valuable insights: EdTech, healthcare, construction, manufacturing, logistics, and so on.
Retail is not an exception.
The competition for customer loyalty is extremely high in this industry. So, retailers are searching for innovative ways to analyze large volumes of consumer data effectively.
A data-first strategy allows companies to understand customers’ buying behaviors, map them to relevant products, identify pain points, and develop marketing strategies to boost sales.
McKinsey analysis of 120+ public consumer and retail companies reveals that those with a mature technology operating model outperform those that operate more traditionally:
“With technology increasingly a competitive differentiator, companies that make software a core part of their organization and harness emerging technologies, such as AI, can lay a strong foundation for sustainable growth.”
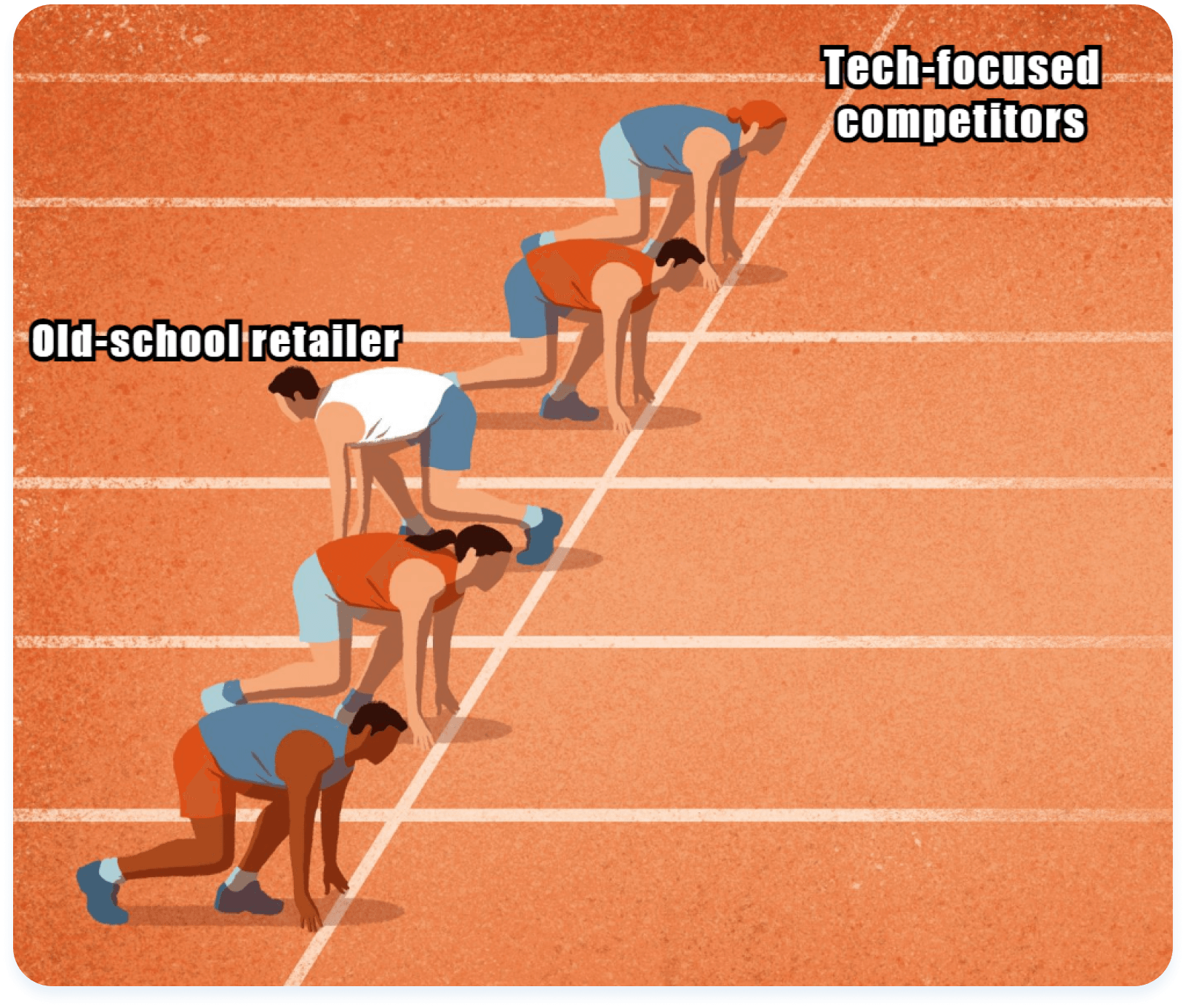
Retailers competition
No wonder 70 percent of the companies said they focus on growing revenue by leveraging AI and related technology.
Let’s remember Walmart, one of the largest retailers, which is undergoing a digital transformation.
Make sense of your data and reap the benefits
We have experts in 15 industries for true data insights.
DS use cases in retail
Now, let’s see how exactly data science helps retailers reduce costs, improve decision-making, and enhance the overall customer experience.
There are various uses of data science (DS) in the retail industry. Let’s dive in and explore them.
Personalization
More than 70 percent of consumers expect personalization and are frustrated when companies don’t deliver it.
Therefore, it’s vital for retail companies to understand customer preferences and behaviors.
With data science techniques and tools, this has become a lot easier.
DS allows companies to segment the customer base, tailor promotions, enhance product recommendations, target ads, improve loyalty programs and retention, forecast the efficiency of offers, and many others.
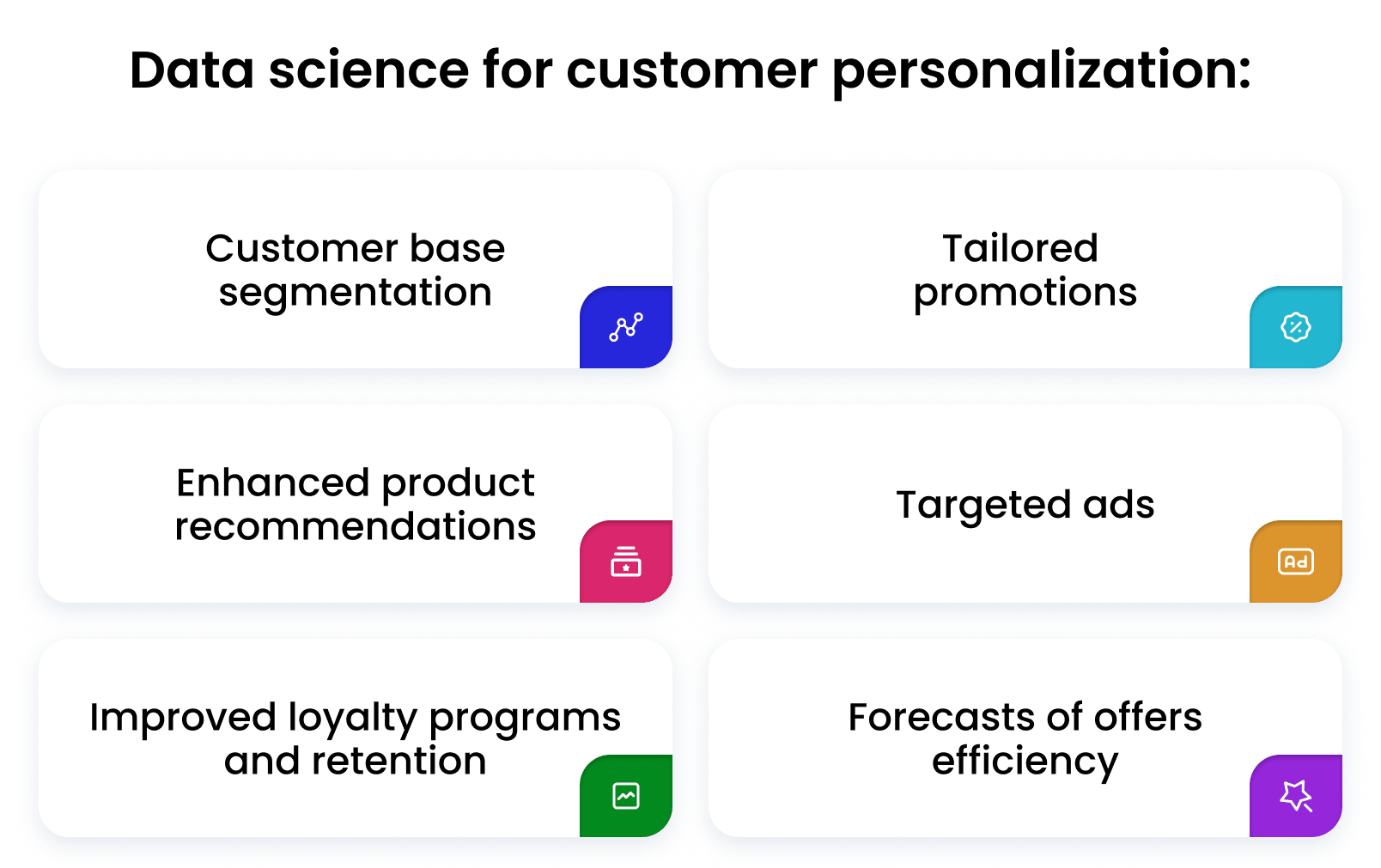
DS for personalization
In general, it helps businesses personalize marketing campaigns, improve customer experience, and drive sales as a result.
Companies that excel at personalization generate 40 percent more revenue from those activities than average players.
The most common examples of successful data science use are Amazon and Netflix.
Netflix analyzes user preferences and habits globally. This allows the service to suggest interesting content for viewers to watch.
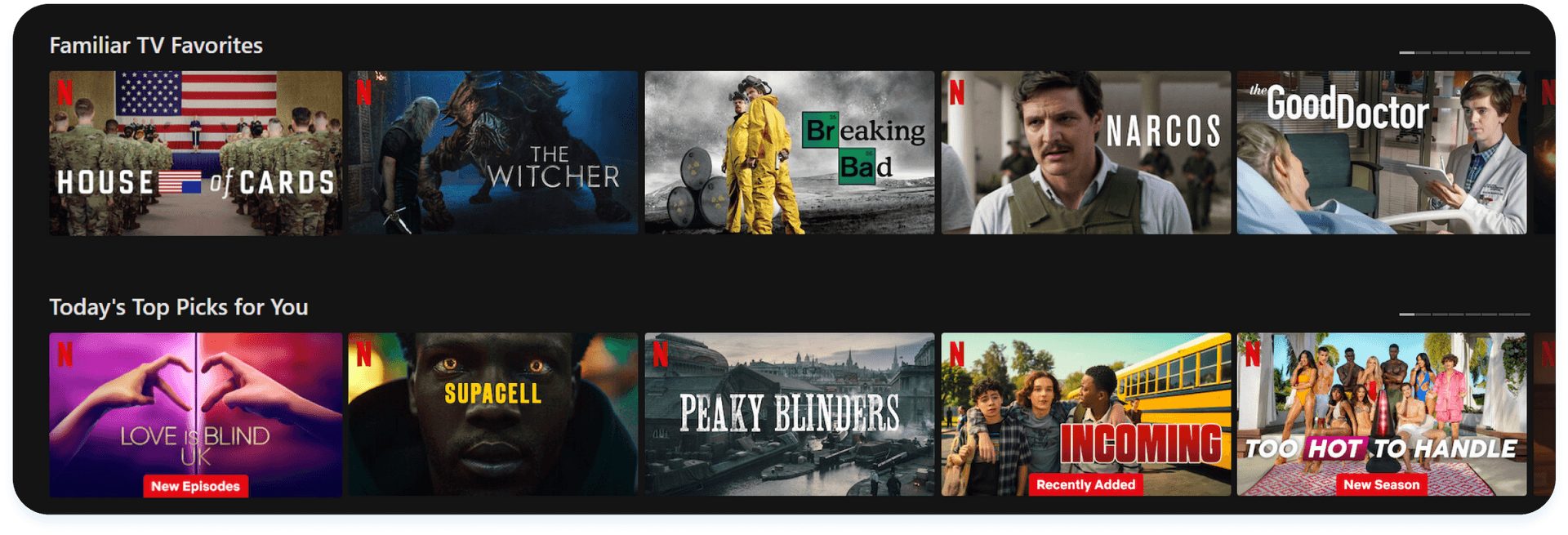
Netflix recommendations
As for Amazon, you can easily search for and add products to your cart, with the platform also providing recommendations for similar items.
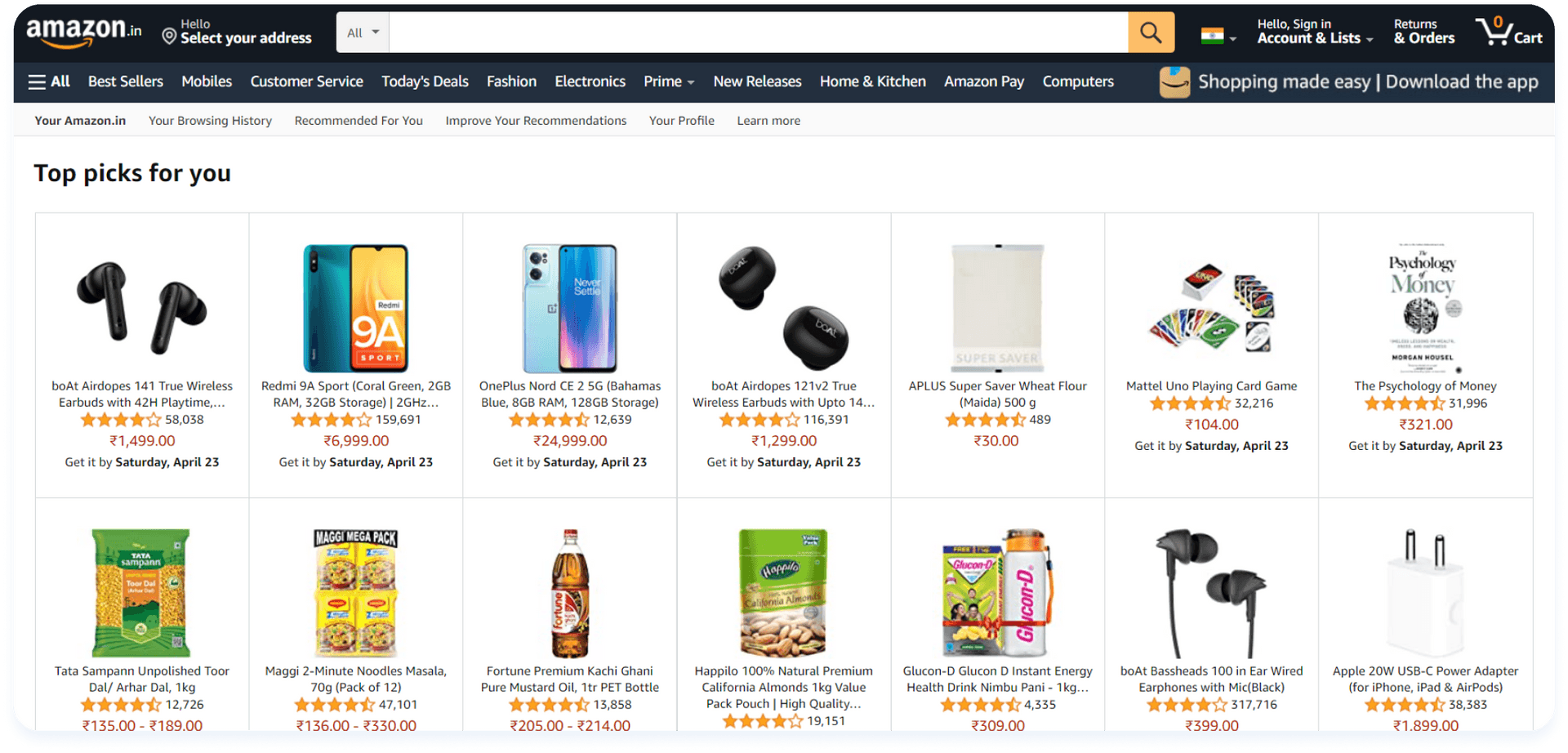
Product recommendations in Amazon
At Aristek, we’ve also helped our clients understand customer behavior better and forecasts the sales for 3 million users
The result: 7% increase in conversions.
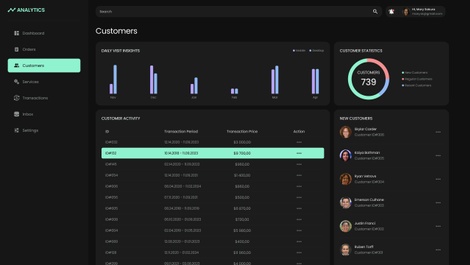
Price optimization
Finding a price point that works for both customers and the retailer is crucial. Pricing decisions are based on a variety of factors:
- Demand;
- Manufacturing costs;
- The average customer’s purchasing power;
- Customer behavior;
- Competitors’ promotions;
- Market trends;
- Other.
What’s more, setting the right prices is driven by precision, attention to detail, and agility, all of which digital pricing transformations (using digital tools and techniques to redesign pricing strategies) facilitate, according to McKinsey.
“Done well, technology-enabled pricing transformations can enhance pricing to generate 2-7 percentage points of sustained margin improvement with initial benefits in as little as 3-6 months.”
Therefore, data science takes price optimization to a whole new level, allowing companies to analyze customer reactions to promotions in real-time, including sales, discounts, and advertising campaigns.
Using regression analysis and predictive modeling, retail businesses can test multiple pricing to forecast demand at different price points for a specific product. It helps them figure out the best rates for different client segments and situations.
Also, dynamic pricing techniques allow retail businesses to modify prices in reaction to shifts in competition, demand, or other variables.
As a result, they optimize sales and profitability while staying competitive in the market.
Common examples that use data science for price optimization are various airlines (e.g., Delta, American Airlines).
They use dynamic pricing algorithms based on factors like demand, time of booking, and historical data.
It enables them to maximize revenue by dynamically adjusting ticket prices in response to market tendencies and customer behavior.
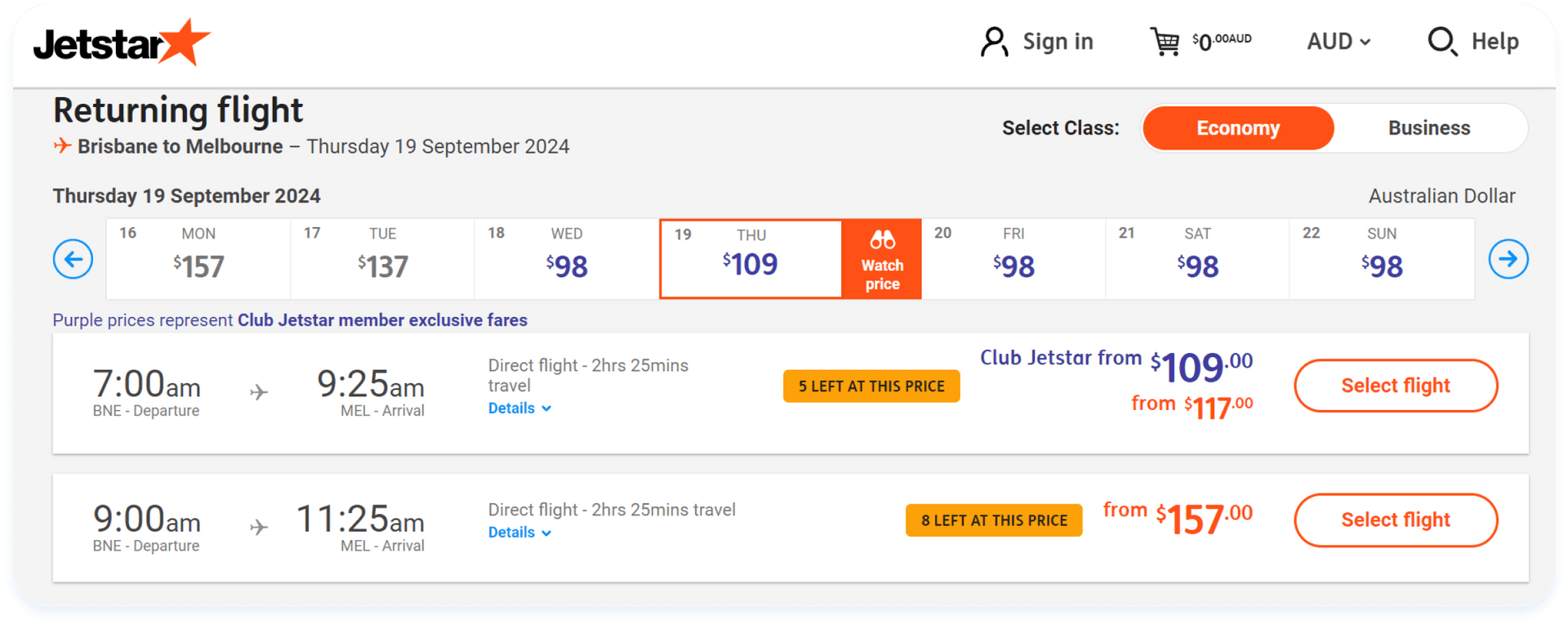
Airlines price optimization
Another great example is Kroger’s mobile app, which uses data science to send customers personalized item recommendations, including prices, for additional insights.
Inventory management
In order to stock up appropriately, retailers try to predict how much of a certain item customers would wish to buy during a specific time frame.
Sales trends are analyzed to identify patterns and develop plans to optimize product delivery and inventory management.
Machine learning (ML) algorithms and data analysis platforms enable retailers to forecast demand and optimize inventory levels, reducing stockouts and overstocking.
What’s more, companies also use demand forecasting to help them store goods in case of emergencies.
For instance, Zara, a global fast-fashion leader, uses data science to optimize inventory management.
By monitoring real-time sales data and customer feedback, Zara adapts production strategies, restocking popular items and minimizing excess inventory for less popular ones.
One more good example: Ralph Lauren and Michael Kors used machine learning to sell fewer garments via markdown prices and get the most of their inventories.
Let’s boost your retail business with cutting-edge solutions
Focus on growing your business. We’ll provide IT support for your plans.
Fraud detection
In the first half of 2024, UK businesses were greatly impacted by an increase in retail fraud.
The analysis of data from NFIB reveals a 545 percent (!) increase in reported cases compared to the same period in 2023.
The financial impact has been severe, with losses soaring to 5.4 million pounds.
According to the global report, organizations lose 5% of revenue to fraud each year.
So, it’s highly important to prevent those losses and also build customer trust. Data science assists well in these goals, too.
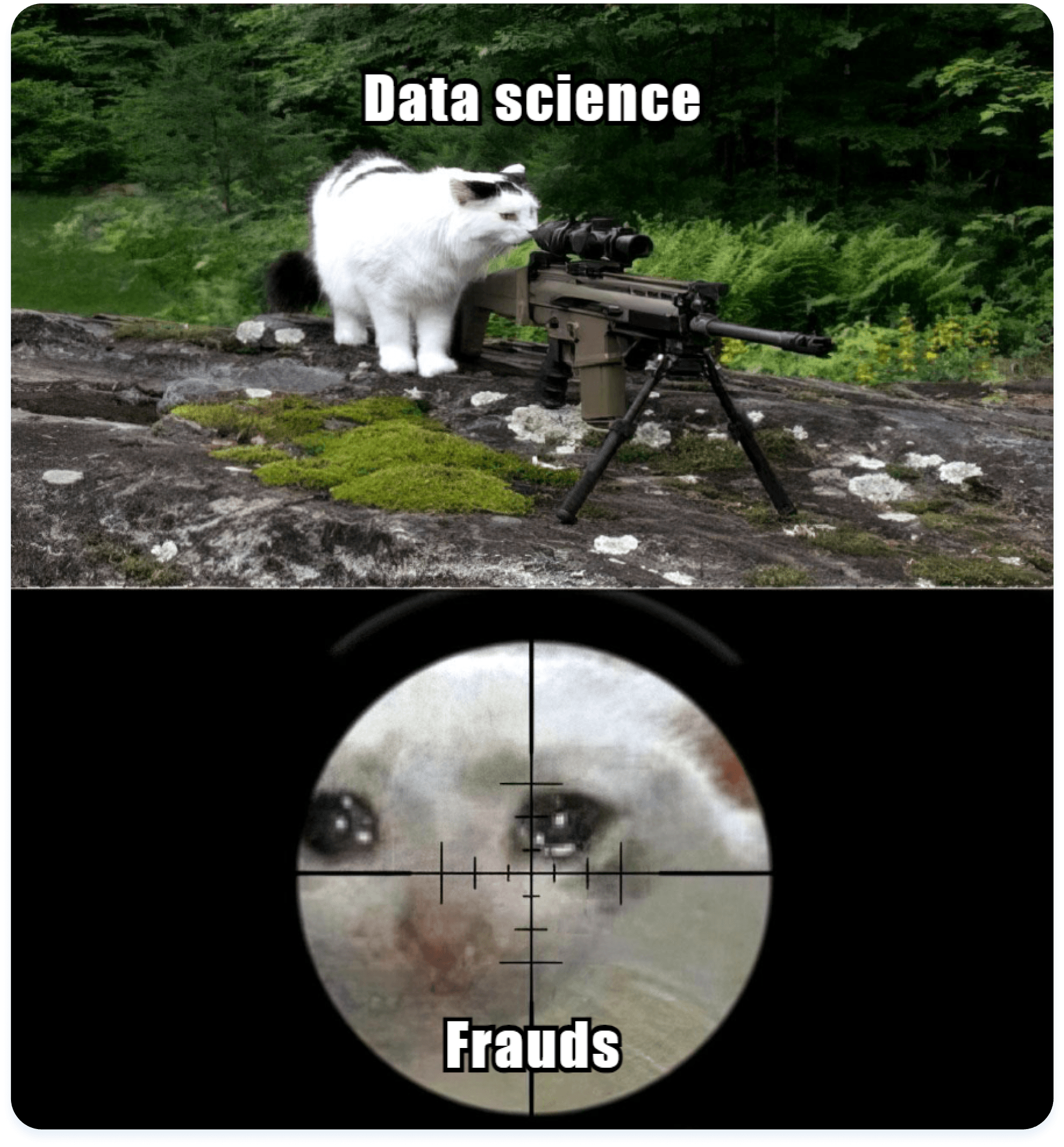
One of the most common frauds are return fraud and credit card fraud.
Return fraud is an online scam type: a person buys an item from a retail store with the intent to return it immediately. They can also use duplicate receipts to get money back.
Credit card fraud is a type of identity theft. It involves unauthorized access to another person’s credit card info in order to charge purchases or withdraw funds from the account.
To detect suspicious activities, DS uses vast amounts of data to analyze operations, user activity, and transactions in real time.
By implementing advanced algorithms and ML techniques, it can quickly identify patterns and anomalies that may indicate fraudulent behavior.
This proactive approach allows organizations to take immediate action to prevent financial losses and protect their reputation.
Moreover, it increases transparency in internal processes, reducing the likelihood of employees participating in fraud.
Supply chain management
Supply chain management is a significant part of the retail operations. It includes demand forecasting, inventory management, route optimization, risk management, and supplier administration.
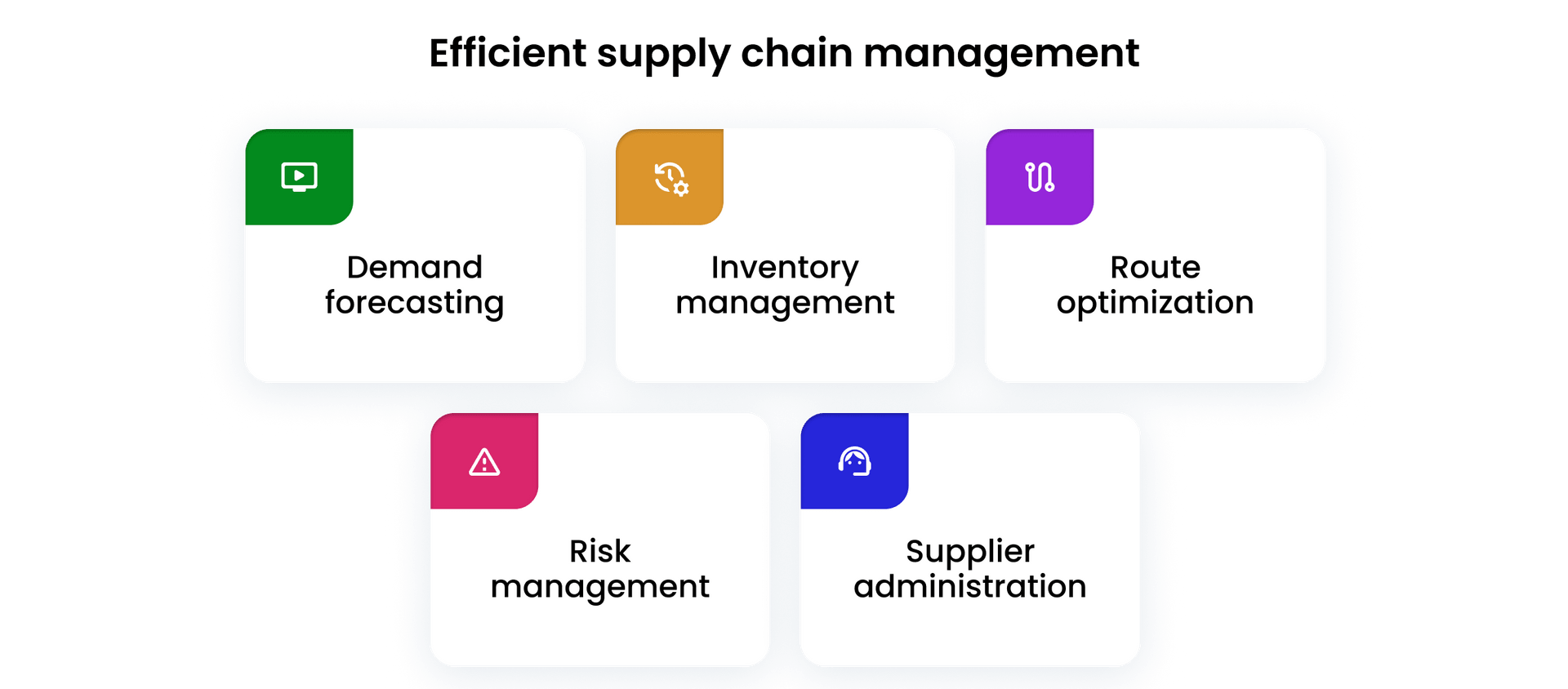
Efficient supply chain management
We’ve already discussed how data science ensures effective demand forecasting and inventory management above.
Let’s see how it helps with optimizing routes, managing risks, and suppliers.
Route optimization
Data science can optimize logistics routes and schedules by considering variables like shipping information, traffic patterns, delivery windows, and meteorological conditions.
As a result, this approach reduces carbon emissions, speeds up deliveries, and lowers transportation costs for retail businesses.
For example, DHL Supply Chain uses data-driven decision-making for route optimization, which enhances their overall productivity and improves decision-making processes.
Risk management
Risk management is crucial for identifying potential hazards in the supply chain and developing backup plans.
According to the Mckinsey report, 83% of respondents experienced at least some raw material shortages over the past year. 90% say that they want to further increase resilience, and almost three-quarters expect to increase the budget allocated to resilience-related actions.
Data science can help identify potential hazards like delays in delivery or commodity flow disturbances, which results in risk reduction.
Also, it ensures business continuity, minimizes output and income loss by streamlining quality control procedures and reducing downtime.
Supplier administration
Data science aids in assessing supplier performance and identifying areas for improvement in supply chain management.
By analyzing data like delivery times, quality indicators, and pricing, data scientists can identify well-performing suppliers.
This enables businesses to enhance supply chain procedures, negotiate better contracts, and improve overall efficiency.
Other use cases
We’ve discussed the most common uses of data science in the retail industry. Naturally, there are more to them.
Some retailers use DS to assist in talent acquisition, merchandising, and choosing a new store’s location.
For example, Starbucks uses data science techniques to select a location for launching a new affiliate based on traffic, demographics, and customer behavior.
Other DS applications include customer lifetime value prediction (CLV), social media trend forecasting, warranty analytics, and so on.
Ultimately, data science in retail can provide valuable insights that help businesses make informed decisions and stay competitive in the market.
AI chatbot for customer support
We developed a chatbot that takes care of routine tasks and helps support specialists handle complex tickets.
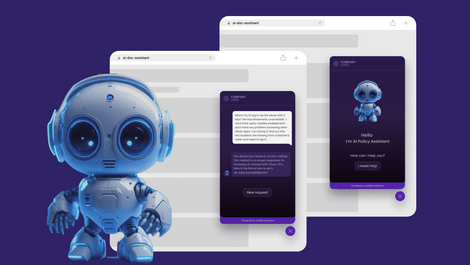
DS benefits in retail
Let’s talk about the benefits retailers get by leveraging data effectively.
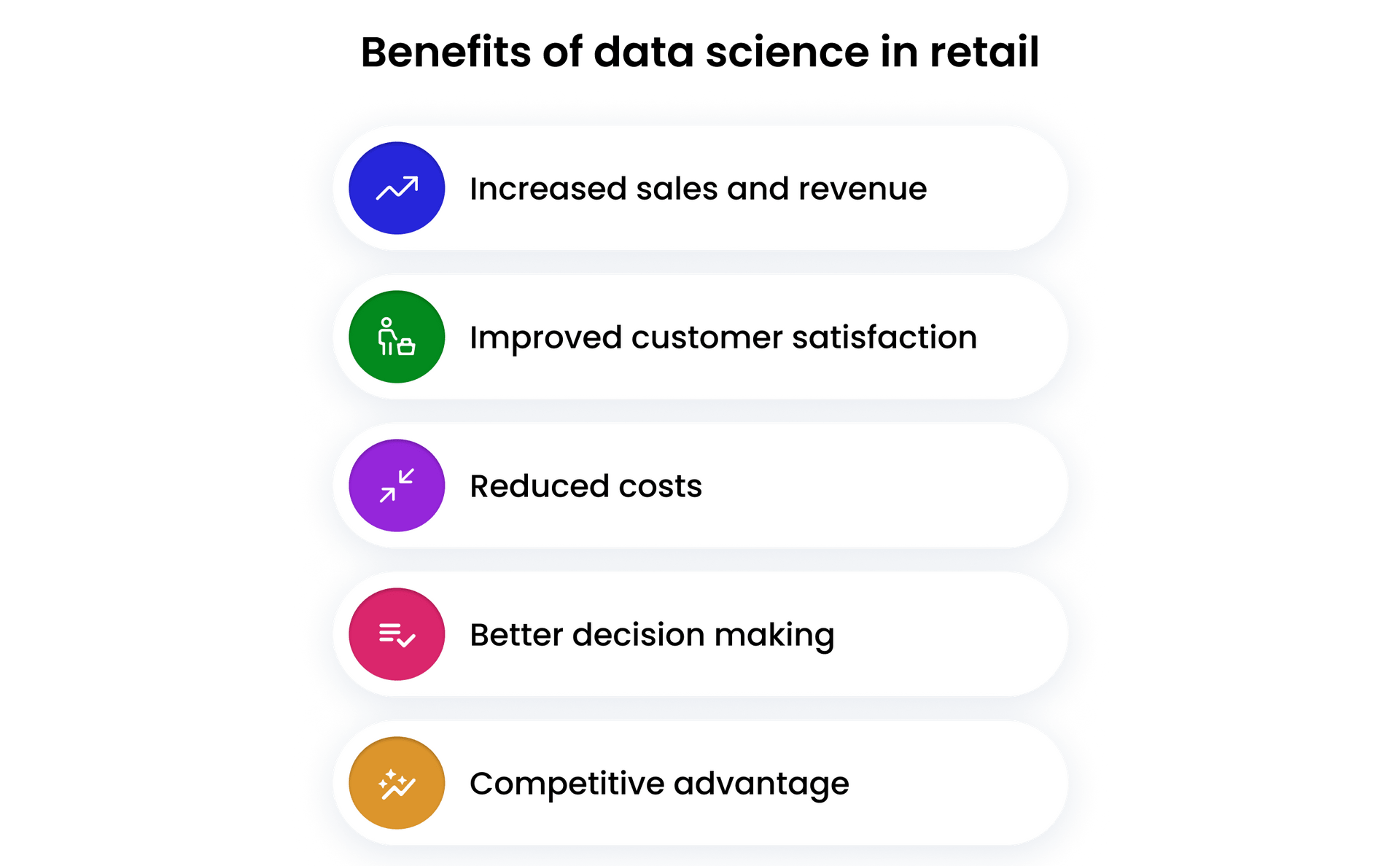
Benefits of data science in retail
Increased sales & revenue
Increased sales and revenue are achieved through targeted marketing strategies and personalized customer experiences.
Additionally, data science helps retailers optimize inventory management and pricing strategies to maximize profitability.
Improved customer satisfaction
Efficient company management, proper stocking of items, and adequate staff can enhance customer satisfaction by ensuring timely and accurate service.
What’s more, better personalization and loyalty programs contribute to customer retention.
Reduced costs
Retail costs can also be reduced thanks to data science. DS has allowed companies to better understand consumer behavior and make more informed pricing decisions.
As a result, customers can now enjoy lower prices on a wider range of products.
Better decision making
By offering specific, fact-based insights, data science revolutionizes decision-making procedures, which leads to more strategic and useful results.
Competitive advantage
The more customers retailers have, the better.
Yet, it is not only quantity that matters, but also quality. Loyal customers drive repeat purchases.
Therefore, data science provides a competitive advantage by boosting customer retention and innovating operational processes.
Tackling DS implementation challenges
No matter how good the benefits sound, retail companies hesitate to implement data science. Let’s talk about the common challenges and solutions to them.
Data quality and security concerns
Processing and analyzing the vast amount of data raises a privacy and security issue. This is because the bigger volumes of data are, the more difficult it is to manage data complexity and protect privacy.
Information theft is a significant security concern, particularly for organizations with sensitive data.
Therefore, with increasing internet exchange, companies must adhere to fundamental data security principles, comply with regulations, and be proactive.
So, what can be done?
Strict access control measures
Sensitive information should only be accessible to the necessary team members or individuals, and the intended use should be identified. For this purpose, role-based access control (RBAC) is enforced.
Additionally, it’s important to review and update access permissions to data on a regular basis.
Robust encryption protocols
Use encryption algorithms to protect sensitive data. Also, store the data on servers and databases to avoid potential breaches and reduce the impact of data theft, even if it occurs.
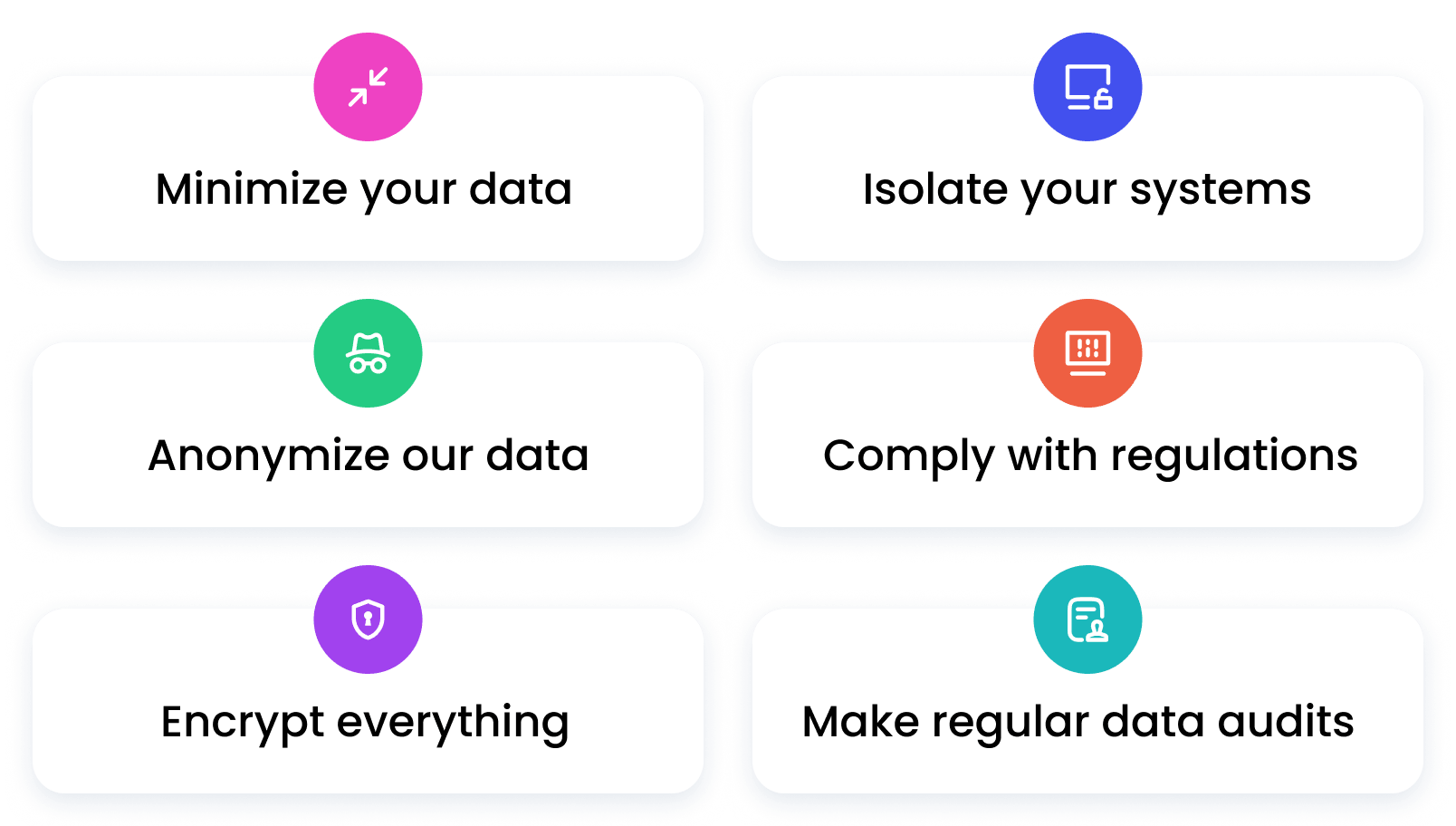
M.A.G.I.C.A: minimize, anonymize, guard (encrypt), isolate, comply, audit
Privacy policy
Develop and enforce clear privacy policies, which outline data collection, processing, storage, and sharing within the organization.
Also, provide employee training on best practices, security protocols, and compliance requirements to foster a data security culture.
These are the first steps you should take to avoid data leaks. For more details, check out this article.
Lack of skilled professionals
Successful data science projects require data analysts and scientists to have problem-solving capabilities and a deep understanding of ML and AI algorithms.
Yet, companies face a talent shortage in data science, struggling to find the right team with in-depth knowledge and domain expertise.
This is because plenty of applicants are underqualified. They simply don’t have relevant data backgrounds. No STEM degree, just a 3-month boot camp certification.
So, they often know the basics, but not much more.
But data science is a real science. To be good at it, strong statistical and math knowledge is needed.
To hire top professionals, reaching out to DS companies with technical expertise and business understanding is a viable option.
Choose a company you can trust and do some background research on the data scientists that will work with you.
Seeking experienced data scientists to share expertise and help with development?
You’re in the right place. Our data scientists have 5+ years of experience and MsS / PhD degrees.
Implementation costs
DS implementation costs include infrastructure expenses, frequent software and hardware upgrades, and managing project scope, which can lead to budget overruns.
Yet, this can be easily tackled with the right approach.
To reduce costs and ensure efficient project management, establish clear objectives, consider cloud services, and implement agile methodologies.
It’s important to encourage team collaboration, monitor metrics regularly, and invest in continuous learning to optimize resource allocation.
Also, most data science projects only take 2-3 months to complete, so they’re not too expensive. You don’t always need a big team, even 2 specialists can be enough if they know your industry.
Finally, not every company needs data science. But if you do, the investments return fast.
Key points
For better understanding, let’s summarize our article in several Q&As.
What’s data science after all?
Data science (DS) involves using various techniques and tools to analyze and interpret complex datasets and extract knowledge from data.
Basically, DS is all about finding insights in data to help make informed decisions.
What is it used for in retail?
There are various use cases of DS in the retail industry. For example, price optimization, inventory management, fraud detection, supply chain management, personalization, and many others.
What are the benefits of using DS in retail?
The benefits include increased sales and revenue, improved customer satisfaction, reduced cost, better decision making, competitive advantage, and so on.
What are the challenges of implementing data science?
The challenges include a lack of skilled professionals, data quality and security concerns, integration with existing systems, and costs of implementation.
How to deal with them?
It depends on the challenge. For example, security issues can be solved with encryption protocols and privacy policy implementation. APIs will be helpful for integration challenges.
As for talent shortages, it’s a good idea to turn to professionals to help you with expertise or hire experts for your project.
Interested in boosting your retail business with data science? Feel free to contact us.
We’ll gladly consult you, develop an innovative solution for your business, or help with complementing your DS team.