Taking an in-depth look at data science in healthcare
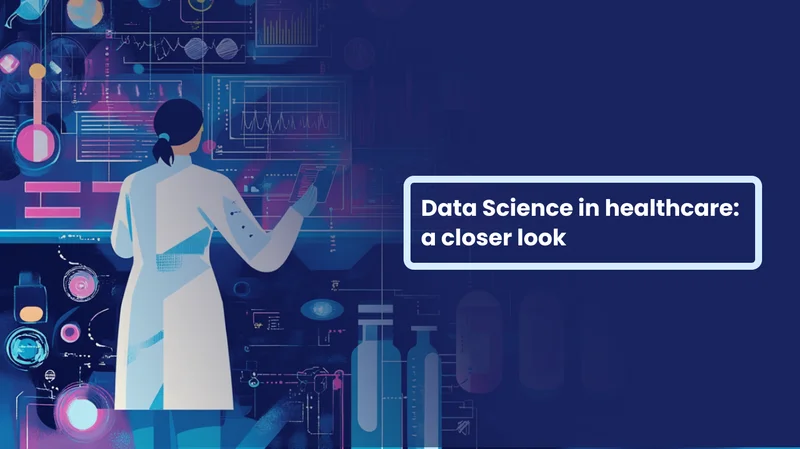
The healthcare services market size has grown significantly in recent years.
Also, it’s expected to grow to $10,908.99 billion in 2028 at a compound annual growth rate (CAGR) of 5.0%.
In this article, we’ll explore how data science (DS) contributes to the development of the healthcare industry by enhancing patient care, improving patient outcomes, and reducing costs.
What’s more, we’ll take a closer look at DS benefits, challenges, and trends.
FYI. Data science (DS) involves using various techniques and tools to analyze and interpret complex datasets.
Its goal is to extract knowledge from data to help make informed decisions.
Not all AI is DS, but there’s a huge overlap. To understand the difference between AI, ML, and data science terms, read this guide first.
What’s data science in healthcare?
A huge amount of data is produced by the healthcare sector. For instance, insurance, treatment plans, patient demographics, test results, and other medical records.
Therefore, data science (DS) offers efficient techniques and tools to make use of it.
Initially, information is gathered from a variety of sources, including the internal systems of medical facilities, claims databases, and Internet of Things (IoT) devices directly.
Then, data scientists process, manage, analyze, and assimilate these large quantities of unstructured data.
As a result, data science provides valuable insights about patients, consumers, and clinicians, allowing organizations to make informed decisions that improve the quality of healthcare.
In general, modern data science technologies make it possible to conduct processes in disease prediction, drug development, medical research, and many other areas more quickly, accurately, and extensively.
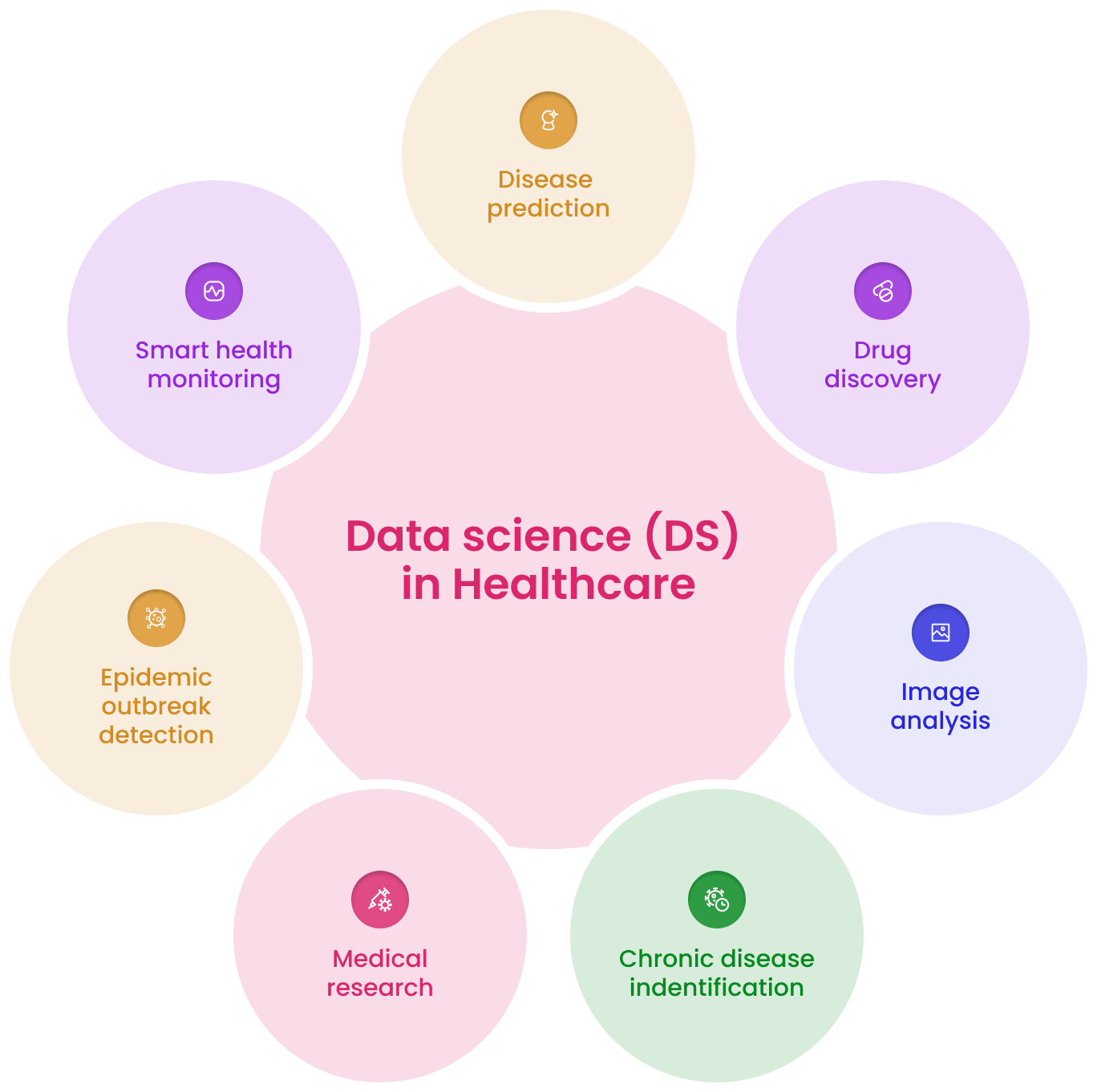
Data science (DS) in healthcare
Benefits of data science in healthcare
There is a lot data science has to offer. We’ve compiled a list of its top benefits in healthcare.
- Improved patient care;
- Early disease prevention and diagnosis;
- Personalized medicine and treatment plans;
- Enhanced new drugs and therapies development;
- Optimized healthcare workflows and resource allocation;
- Reduced healthcare costs and staff workload;
- Increased overall efficiency.
Let’s probe deeper and explore data science advantages and use cases in healthcare.
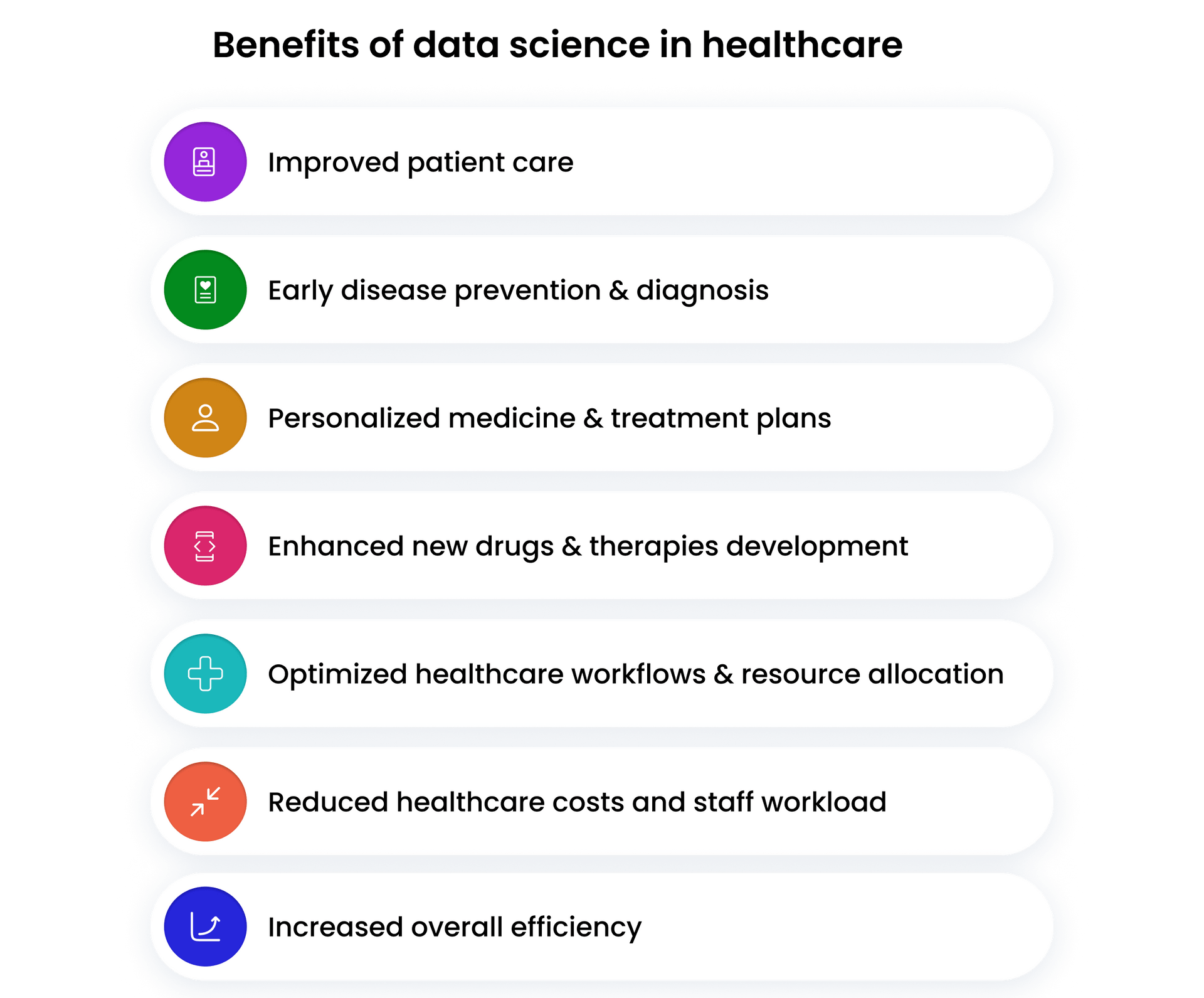
Benefits of data science in healthcare
Better patient care
Hospital readmissions are considered to be one of the most important issues that influence the quality of patient care.
Research shows that 15.3% of 491 patients were readmitted within a month of their discharge. The most common cause was persistence of symptoms (43%).
To deal with this issue, DS assists in identifying at-risk patients and providing specialized follow-ups to ensure that nobody is discharged until they’ve been completely cured (and nobody stays in the hospital longer than needed).
Also, using predictive analytics, it’s possible to forecast and prepare for patient readmissions based on their historical data, patient profiles, and treatment outcomes.
Therefore, data science leads to reductions in readmission rates, hospital stays, and healthcare costs.
Looking for data expert advice?
Book a free call with an experienced data science consultant with 14+ years of expertise.
Early disease prevention & diagnosis
Logistic regression and neural networks are used for early disease detection, which results in enhanced diagnostic accuracy, early intervention rates, and better patient outcomes.
Also, these machine learning (ML) techniques analyze patient data to identify patterns and predict the likelihood of disease development, allowing for timely and targeted medical interventions.
Providers and payers for care also use ‘population health’ ML models to even predict populations at risk of particular diseases or accidents.
For example, Cancer Epidemiology, Biomarkers & Prevention research showed that a predictive analytics model was able to accurately identify patients at higher than normal risk for pancreatic cancer.
By identifying the high-risk population, doctors can improve prevention efforts and early screenings.
Personalized medicine & treatment plans
According to research, machine learning algorithms can read clinical charts quicker and more correctly than human review methods, enabling plans and providers to detect hidden risk factors and healthcare gaps, increase risk score accuracy, and make informed choices for better patient care.
That’s why using ML algorithms, such as decision trees and random forests, to tailor treatment based on patient data leads to enhanced treatment efficacy, reduced adverse reactions, and patient satisfaction.
Enhanced new drugs & therapies development
Data science presents numerous opportunities to increase the efficiency and speed of the discovery process.
For example, ML algorithms are used to find and predict the effectiveness of potential medication candidates.
This is achieved by analyzing large amounts of data, such as genetic information, clinical trial data, and molecular structures.
Optimized healthcare workflows & resource allocation
Through the analysis of patient flow, use of resources, and wait times data, DS approaches can boost healthcare operations.
By optimizing scheduling, staffing, and resource allocation, this data eventually improves patient care and lowers expenses.
As a result, organizations reduce operational costs, improve patient throughput, and increase resource utilization.
Overcoming data science challenges
Data science can really take healthcare organizations to a new level. Yet, there are still two main concerns. Let’s resolve them together.
Data privacy
When it comes to processing large amounts of data, organizations should always consider data privacy.
This is because the bigger the volumes are, the more difficult it is to manage data complexity and protect privacy.
Data leakage is a significant security concern, especially for healthcare organizations with sensitive data.
Therefore, with increasing internet exchange, companies must adhere to fundamental data security principles, comply with regulations, and be proactive.
Enforce strict access control measures
Identify the intended use and allow access to sensitive information only to the necessary team members or individuals. For this purpose, role-based access control (RBAC) is enforced.
Also, don’t forget to review and update access permissions to data every now and then.
Implement robust encryption protocols
Using encryption protocols is a great way to protect sensitive data. You can also store the data on servers and databases to avoid potential breaches and reduce the impact of data theft in case it occurs.
What else can be done? Follow the M.A.G.I.C.A principle!
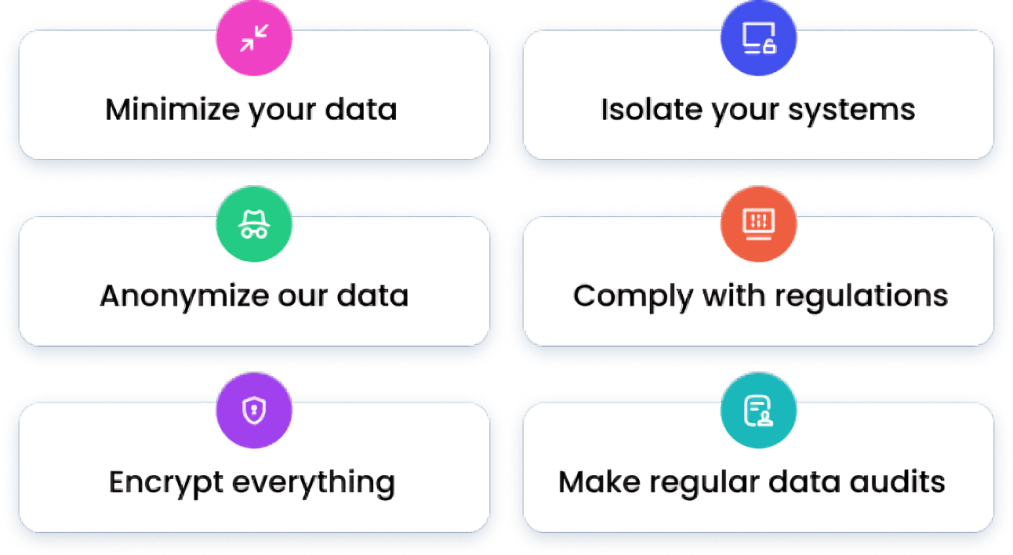
M.A.G.I.C.A: minimize, anonymize, guard (encrypt), isolate, comply, audit
Create a privacy policy
Create a clear privacy policy, which outlines the procedures of data collection, processing, storage, and sharing within the organization.
Organize staff training on best practices, security protocols, and compliance requirements to foster a data security culture.
These are the first steps you should take to avoid data leaks. To learn more, check out this article.
Data accuracy
How to ensure the results are accurate and you can trust those insights?
The answer is pretty simple — choose true professionals. This is because data science is a real science.
To be good at it, strong statistical and math knowledge is needed.
Lots of applicants are underqualified. They simply don’t have relevant data backgrounds. No STEM degree, just a 3-month bootcamp certification.
That’s why organizations face a talent shortage in data science, trying to find the right team with in-depth knowledge and domain expertise.
To find top professionals, reach out to data science companies with technical expertise and business understanding, do some background research on the data scientists who’ll work with you, and select a vendor you can rely on.
Seeking experienced data scientists to share expertise and help with development?
You’re in the right place. Our data scientists have 5+ years of experience and MsS / PhD degrees.
The future of data science in healthcare
Using big data to support precision medicine is one of the top trends in healthcare data science.
This is because big data analytics allows healthcare to examine huge databases, including genetic information, clinical records, and lifestyle data, to adapt treatments and interventions to specific patients.
The big data in healthcare market size was valued at USD 25.1 billion in 2023 and is projected to grow from USD 29.58 billion in 2024 to USD 82.19 billion by 2032.
Another big trend is remote patient monitoring technology in wearable devices. It provides real-time data for better decision-making and early intervention.
Integrating this data into electronic health records (EHR) will also reduce in-office visits.
Hybrid platforms are also on the rise. This means that businesses are combining their analytics and data science resources by developing a platform.
This strategy enables creating a big data analytics center with technologies designed to handle and process every stage of the data science life cycle.
Summing up
Data science isn’t just a buzzword in the healthcare industry. It does make a change.
Its benefits include better patient care, early disease prevention, personalized medicine, optimized healthcare workflows and resource allocation, reduced healthcare costs, and improved efficiency.
If you’re thinking about using data science, this is your sign. Contact us to learn more.